Mastering Line Graph Creation with Ease
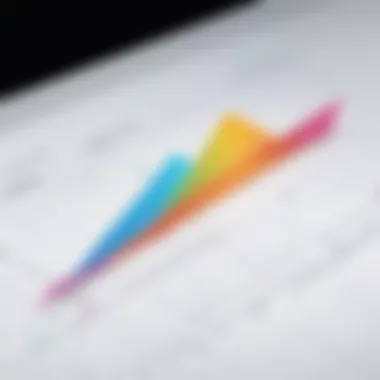
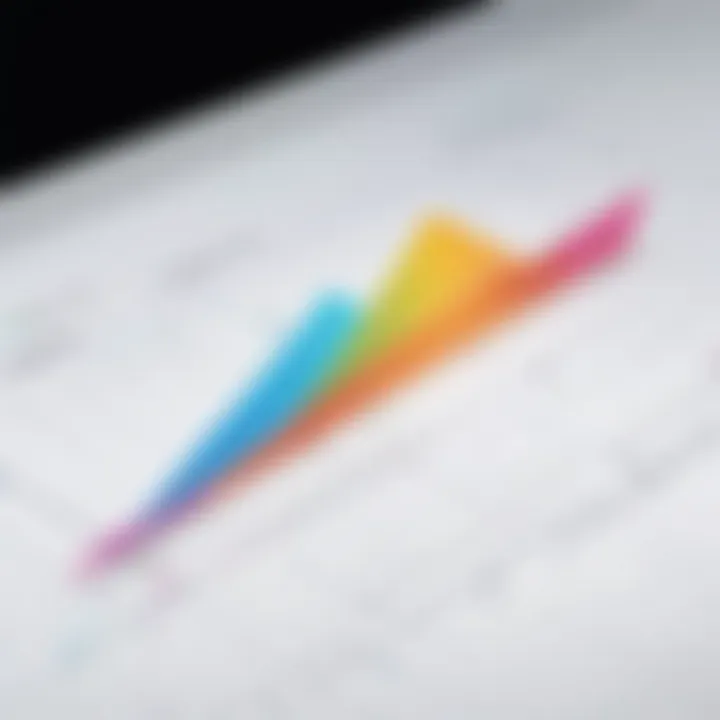
Intro
Creating line graphs is more common than one might think, and the simplest ways to do this can make a world of difference in data representation. Line graphs serve as powerful tools in visualizing trends over time, relationships among variables, and changes in data sets. In this section, we will briefly touch on why understanding line graphs is essential for professionals and enthusiasts alike.
To start, line graphs take data points and connect them with a straight line, making it easy to see fluctuations and overall trends at a glance. For business analysts, this clarity can help in making well-informed decisions. Students can also benefit from this, as solid skills in depicting data graphically can boost their academic performance and critical analysis abilities. It's a bit like having a well-organized toolbox; everything you need for effective data analysis is right at your fingertips once you know how to build and read line graphs.
"A good graph is worth a thousand words."
This adage rings true; the better your graphs, the easier it is to communicate results and insights to your audience. We’ll explore the nuts and bolts of constructing line graphs, emphasizing simple techniques and tools that anyone can use.
With a little finesse and understanding, anyone can transform their raw data into visual masterpieces that tell stories in ways numbers alone cannot.
Understanding Line Graphs
In the realm of data visualization, line graphs pop up like daisies in spring. They serve as a bridge connecting raw numbers and human comprehension. This section emphasizes the importance of grasping the concept of line graphs, which are not merely visual aids but powerful tools for interpreting and communicating complex data sets. Understanding line graphs can transform how we analyze trends over time, helping to spotlight significantly meaningful patterns that might otherwise go unnoticed.
Definition and Purpose
A line graph is essentially a visual representation that depicts data points in a temporal manner. By plotting data points on a coordinate system, these graphs illustrate how a dependent variable changes in relation to an independent variable. Generally, the x-axis represents time, while the y-axis showcases a variable being measured. The primary purpose of using a line graph is to highlight trends and fluctuations in data over specific intervals, providing a clear picture of how something evolves.
Common Applications
Line graphs find their way into various fields, from academia to business analysis and even in everyday life. For instance, educators often use them to illustrate students' performance over terms, while businesses leverage them to track sales growth. Additionally, they're popular among scientists for displaying experimental results or changes in environmental data. The versatility of line graphs makes them indispensable across disciplines.
Key Components
Understanding the foundational elements of a line graph is pivotal. Each component plays a significant role in ensuring the graph communicates information effectively.
Axes
The axes are akin to the backbone of a line graph. They provide the framework that gives meaning to the data presented. Usually, the x-axis runs horizontally, while the y-axis extends vertically.
- Key Characteristic: The axes allow for accurate data representation, enabling viewers to quickly assess values in correlation to time or categories.
- Why It’s Beneficial: A well-defined axis aids in clarity, reducing confusion and enhancing the graph's overall interpretability.
- Unique Feature: Axes often display scales that can be linear or logarithmic, each having its pros and cons depending on the nature of data being analyzed. For example, while linear scales are easy to read and understand, logarithmic scales can better represent data that spans several orders of magnitude.
Data Points
Data points mark the individual values plotted on the graph. They portray the relationship between the two variables being analyzed.
- Key Characteristic: Each point represents a specific observation at a given time or condition, establishing a visual narrative of trends.
- Why It’s Beneficial: Data points provide the essential landmarks that facilitate quick insights into the data set.
- Unique Feature: Different styles of data points, like circles or squares, can be used to indicate whether they are estimates or actual findings, which can either add clarity or cause confusion based on how it’s handled.
Legend
The legend acts like a map key, explaining the symbols and colors used in the graph. It is crucial, especially in line graphs that represent multiple data sets.
- Key Characteristic: The legend simplifies the interpretation, allowing viewers to distinguish between various lines clearly.
- Why It’s Beneficial: Having a legend helps prevent miscommunication or misunderstanding, especially in graphs where several variables are displayed.
- Unique Feature: A well-designed legend can significantly enhance the graph's clarity, making it easy for someone to decipher at a glance what each line represents, which can greatly improve decision-making when analyzing data.
Understanding the critical components of line graphs—axes, data points, and legends—is the first step in mastering this tool. With these elements, users can not only create graphs but also derive meaning from them, improving their data analysis capabilities. This understanding lays the groundwork for more advanced techniques and practices discussed in upcoming sections.
Types of Data Suitable for Line Graphs
When it comes to visualizing data, understanding which types of data are suitable for line graphs is crucial. Line graphs excel in illustrating trends over time or quantifying relationships between variables. In this section, we'll dive into the specifics of quantitative data and temporal data, which are the bedrock upon which effective line graphs are built.
Quantitative Data
Quantitative data consists of numerical values that can be measured. This type of data provides the foundation for creating a line graph, as it allows for mathematical operations and comparisons.
Consider the example of tracking sales figures over several months. Each month's sales can be plotted on the Y-axis, while the months of the year can be denoted on the X-axis. By connecting the dots between each data point, you create a visual representation that makes discerning trends over time much more straightforward. This allows stakeholders to pinpoint periods of growth or decline at a glance, rather than wading through spreadsheets filled with figures.
The key benefits of utilizing quantitative data in line graphs include:
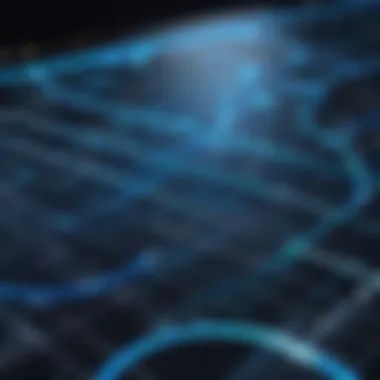
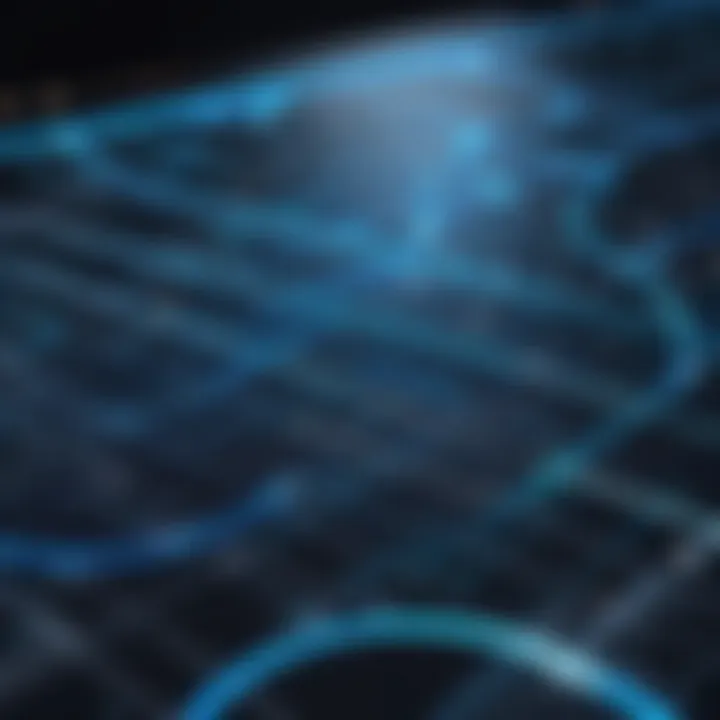
- Ease of interpretation: Quickly assess increases or decreases in data.
- Comparative insights: Spot correlations between different data sets when multiple lines are included.
- Effective monitoring: Keep track of progress towards targets, helping you make informed decisions based on trends.
Temporal Data
Temporal data is another crucial component typically represented on line graphs. This falls under the umbrella of data that is time-based. In simple terms, it answers the "when" questions. For instance, if you're measuring average temperature changes throughout a year, each month would be represented distinctly on the X-axis while the temperature values would occupy the Y-axis. As time progresses, a continuous line charts the fluctuations, offering vivid insights into seasonal changes.
Using temporal data in your graphs provides numerous advantages:
- Trend tracking: Understand seasonal patterns or fluctuations across specified intervals.
- Forecasting: Help predict future outcomes based on historical data trends.
- Data storytelling: Engage an audience better by illustrating relatable timelines.
Overall, choosing the right type of data to plot in line graphs is paramount for effective data communication. When appropriate quantitative and temporal data is utilized, line graphs become powerful tools for monitoring, predicting, and conveying significant trends effectively.
"Data is the new oil, and a line graph is a valuable refinery that helps turn crude information into insightful narratives."
This emphasis on the correct data types not only enhances clarity in your graph but also boosts its effectiveness in communicating your message.
Tools for Creating Line Graphs
Creating effective line graphs involves not just data but also the right tools. This section delves into various tools available for constructing line graphs, emphasizing how they can streamline the process and enhance the quality of visual representations. The choice of tools can make the difference between a graph that communicates well and one that obfuscates data. Therefore, understanding the different options can aid users in selecting the most appropriate ones for their needs.
Software Options
Spreadsheet Applications
Spreadsheet applications, like Microsoft Excel or Google Sheets, are cornerstones in data analysis and visualization. They allow users to enter data in an organized manner, making it simple to create line graphs from structured datasets. A key characteristic of spreadsheet applications is their user-friendly interface, which makes it easy for novices and experts alike to generate graphs without much hassle. These platforms automatically generate graphs as users input or adjust data, thus providing immediate visual feedback.
One particularly unique aspect of spreadsheet applications is the ability to update graphs in real-time. As a user modifies data in a cell, the graph instantly reflects those changes. This live updating feature is a significant advantage, especially for tasks that require frequent adjustments or presentations that evolve over time. However, users should be mindful that while spreadsheet applications are powerful, they can sometimes struggle with larger datasets, leading to slow performance or crashes.
Data Visualization Tools
Data visualization tools, such as Tableau or R, offer advanced options for creating line graphs. These platforms are renowned for their powerful analytical capabilities, enabling users to explore complex datasets in depth. The key characteristic that sets these tools apart is their emphasis on interactivity; users can manipulate data points, zoom in on specifics, and generate dynamic graphs in a way that traditional spreadsheet applications may not allow.
One of the unique features of these tools is the ability to work seamlessly with different data sources. Whether it's pulling information from databases, cloud services, or spreadsheets, users often find flexibility in data connections. This can greatly streamline the data collection process. Although they offer robust options, some learning curve is involved, which could be a downside for beginners who might find initial navigation daunting.
Online Graph Generators
Online graph generators, like Canva or Google Charts, provide a quick and accessible way to create line graphs without needing specialized software. This characteristic of being web-based is particularly appealing; users can access these generators from anywhere as long as they have internet connectivity. They often come with pre-set templates, making it easy to generate visually appealing graphs with minimal effort.
A standout feature of online graph generators is their ease of sharing and embedding capabilities. Users can simply create a graph and share a link or embed it within websites or presentations. While offering great convenience, they do come with limitations in data handling. Users may find these platforms less effective for larger or complex datasets, which might require additional work to format properly.
Hardware Considerations
When creating line graphs, it's important to consider the hardware in use. While software plays a pivotal role, the hardware on which these programs operate can impact performance significantly. For instance, older computers may struggle with running sophisticated data visualization software or may become sluggish when managing large datasets. It's crucial for users to be aware of their device's specifications to ensure smooth operation. Additionally, for those working on collaborative projects, having a reliable internet connection is vital, especially for cloud-based platforms, where slow speeds can hinder progress or lead to frustrating delays.
Step-by-Step Guide to Creating a Line Graph
Creating a line graph may sound straightforward, but there’s a delicate art to it that can significantly influence data representation. This guide aims to walk you through the essential steps, ensuring that your journey from raw data to a polished graph is both efficient and effective. By breaking down the process, you can easily comprehend each crucial element involved in constructing a line graph, enhancing the clarity and usability of your visual data.
Data Collection
Before you even think about that bright, shimmery line graph, the first order of business is data collection. Gathering the right data sets the foundation for your graph. If you're tracking sales over the months, ensure you have accurate figures for every month you wish to present. The more reliable the data, the more credible your line graph will appear. Moreover, think about the source of your data. Whether it comes from a trusted database or a recent survey, make sure it adheres to good practices.
- Importance of Accuracy: Ensure that the figures reflect what they are supposed to, devoid of errors. Inaccurate data can lead to misleading representations.
- Relevancy: It’s crucial to gather data that is relevant to your specific aim. Ask yourself what story you want the graph to tell, and collect accordingly.
Data Organization
Once you've collected your data, organizing it appropriately is the next step. It’s not just about having numbers; you want to arrange them in a way that’s coherent. Perhaps you’re using a spreadsheet or a piece of software—whatever your choice, structure matters.
- Creating a Clear Table: Your data should be laid out clearly, maybe in columns and rows. Each column can represent different variables—such as time, quantity, and anything else critical to your analysis.
- Labeling: Make sure to label each column clearly so that the purpose of your data becomes evident. Labels act as signposts, guiding viewers through what they're looking at.
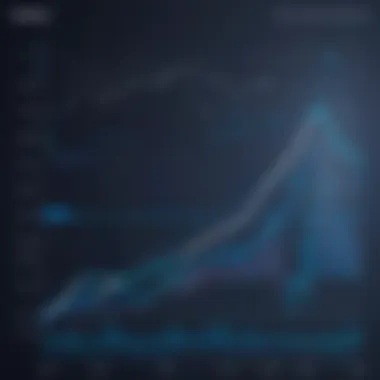
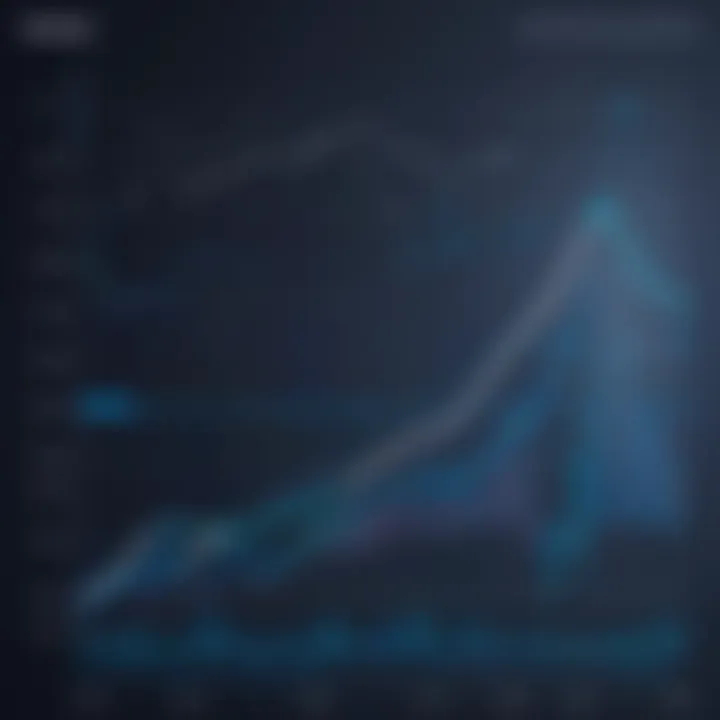
Designing the Graph
When it comes to designing, it's about making the graph visually accessible and informative without overwhelming the viewer. This is where the real creativity combines with data.
Selecting Axes
Selecting axes is paramount. The x-axis usually represents the independent variable, while the y-axis typically showcases the dependent variable. A thoughtful selection of axes directly contributes to the graph's readability.
- Key Characteristic: The axes should correspond well with the data being represented. For example, if you're tracking temperatures over a time period, your x-axis should clearly reflect the dates.
- Why It’s Beneficial: A well-chosen axis layout can drastically improve how the audience interprets the data. The clearer the axis, the better the overall understanding.
- Unique Feature: Pay attention to the scale. A well-thought-out scale can make a difference in how trends are viewed. Use logarithmic scales for large ranges, or linear scales for uniform data ranges.
Plotting Points
Once the axes are set, it's time to plot the data points. This involves placing corresponding values correctly along the axes you’ve just established. This may seem a tad simple, but accuracy is everything.
- Key Characteristic: Each point should represent a specific piece of data. If you’re plotting monthly sales, each point corresponds to a month and its respective sales figure.
- Why It’s Beneficial: Properly plotted points ensure that patterns can be easily visualized and analyzed by viewers. An accurate representation allows for quick comprehension and insight generation.
- Unique Feature: Consider using markers such as dots or X’s. While line graphs typically feature a continuous line, choosing explicit markers helps to delineate individual data points, aiding clarity.
"A graph should tell a story at a glance; the clearer the data points, the easier it is for viewers to derive insights."
Creating a line graph is more than just connecting dots. It’s about weaving a narrative through data. Following these steps carefully not only ensures accuracy but also enhances the ability to meaningfully convey information through visual representation.
Customizing Your Line Graph
Customizing a line graph is a vital step that can significantly enhance its effectiveness. While creating a line graph involves plotting data points and connecting them with a line, customizing it can make the information more digestible and visually appealing. Customization not only aids in presenting data clearly but also influences how the audience interprets the information. With a well-thought-out design, your line graph can stand out, making complex data more approachable and easier to understand.
Color Schemes and Styles
The choice of colors in your line graph is not merely a matter of aesthetics; it also plays a crucial role in conveying information efficiently. A well-chosen color scheme can help different data sets stand apart and allow the viewer to distinguish between them without much effort.
- Consistency: Use colors that match your overall theme or branding. Consistency can help reinforce your message and make your graph more professional.
- Contrast: Ensure that there’s a clear contrast between the different lines and the background. This helps viewers quickly recognize trends or changes in data.
- Accessibility: Consider colorblind-friendly palettes. Not everyone perceives colors the same way, so lean towards shades that are easily distinguishable by most. Tools such as ColorBrewer can assist in selecting appropriate palettes.
Implementing styles, like using dashed or dotted lines to denote variations between datasets or applying different markers for specific data points, can enhance understanding. For example, you might use solid lines for actual data and dashed lines for projections. This differentiation is crucial during presentations or reports.
Adding Annotations
Annotations on line graphs serve as guideposts that provide context. They tell a story that the raw data alone often cannot convey. Simple markers or notations can alert viewers to important events or noteworthy changes in data. Here are some beneficial approaches:
- Highlight Key Events: If your data represents time series, you can annotate significant occurrences that may correlate to peaks or troughs in your graph. For instance, if sales surged during a holiday season, mark it clearly with an arrow and brief description.
- Explanatory Notes: Sometimes, adding a note explaining an anomaly can prevent misinterpretation. For example, if there was a sudden drop in user engagement, an annotation can explain that it resulted from a system outage.
- Interactive Elements: If you're creating a digital graph, consider adding tooltips or pop-ups that reveal more information when the cursor hovers over a data point. This adds another layer of interactivity that can keep the audience engaged.
"Customization isn’t just about looking good; it’s about making information accessible and understandable."
Ultimately, the aim is to ensure that your line graph communicates effectively, making the complex simple. Remember, when it comes to data visualization, clarity is key.
Best Practices for Line Graphs
Creating a line graph is not always as straightforward as it might seem, and applying best practices can make a significant difference in clarity and precision. When it comes to expressing data visually, it’s easy to stumble into pitfalls that could mislead an audience. Therefore, understanding these best practices is paramount for anyone looking to present data effectively.
Data Clarity
When it comes to line graphs, clarity reigns supreme. A clear presentation of data allows viewers to digest the information without squinting at cluttered visuals. Important factors to consider for achieving this clarity include:
- Simplified Design: Keep the design simple. Avoid the temptation to use intricate patterns or excessive grid lines which can create visual noise. It’s advisable to use solid, contrasting colors for the lines. The goals here are readability and straightforwardness.
- Labels and Legends: Properly label your axes and include a legend if multiple lines are being represented. Viewers should not have to guess what the data signifies. Well-placed and clear labels help in preventing ambiguity.
- Data Points: Don't clutter the graph with unnecessary data points. Instead, focus on essential points that tell the story of the data being represented. A good rule of thumb is to maintain a balance between definitive markers and general trends.
By taking the time to refine these aspects, the audience is better able to engage with the data, which in turn enhances their analytical capabilities.
Avoiding Misrepresentation
Misrepresentation of data isn't just frustrating; it can also lead to erroneous conclusions and decisions. To avoid this, consider the following practices:
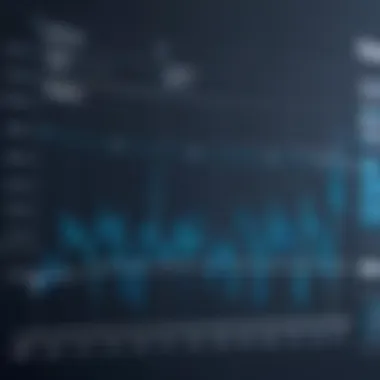
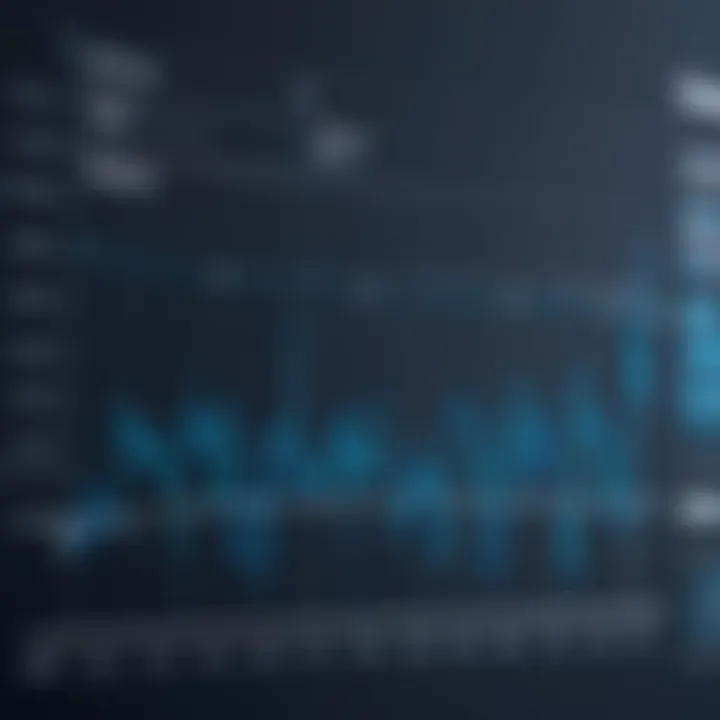
- Choosing Appropriate Scale: The scale of the axes should be chosen with careful deliberation. A manipulated scale can dramatize changes or trends which might not be as significant when viewed accurately. Ensure the scale reflects true changes in the data.
- Accurate Data Representation: Always ensure that the plotted points accurately represent the data collected. This means double-checking calculations and confirming that no data is missing or misrepresented. Merging data sets without confirmation can create misleading visuals.
- Contextualizing Data: Provide context for the data. This means explaining what the graph is meant to convey and where the data comes from. Without context, even accurately plotted data can be misunderstood or misapplied.
Following these best practices not only enhances the effectiveness of the line graphs you create but also contributes to a more ethical approach to data presentation.
"In the age of information, clarity is power. Presenting data clearly ensures that your message is received loud and clear."
Adopting these best practices can elevate your line graphs from routine to remarkable, making your data not just seen, but understood.
Troubleshooting Common Issues
Creating line graphs can sometimes feel like sailing in uncharted waters. Just when you think you have the data plotted correctly, issues arise that can make the final visualization confusing or even misleading. By understanding how to troubleshoot these common problems, users can enhance the clarity and reliability of their graphs. Addressing these issues not only helps in refining the data display but also fosters a deeper comprehension of data trends and relationships.
Data Overlap
One of the prevalent challenges when crafting line graphs is data overlap. This occurs when multiple data points or lines occupy the same space on the graph, particularly in cases where several datasets are depicted together. A classic example is when tracking the performance of several products over the same timeline, the lines may crisscross, making it difficult to differentiate between the datasets.
To tackle data overlap, consider the following strategies:
- Choose contrasting colors: Opt for colors that stand out against each other. For instance, if one line is blue, consider using an orange or green for another dataset.
- Increase line thickness: Thicker lines can help distinguish between datasets. Just ensure you don’t go overboard, making the graph messy.
- Add markers: Dot the lines with distinct markers at each data point. This allows viewers to easily identify where one line ends and another begins.
In scenarios where the datasets cannot be easily separated, perhaps due to the nature of the data, it might be more effective to use a different type of graph, like a scatter plot.
Inaccurate Axes Scales
Another frequent culprits in the realm of line graphs is inaccurate axes scales. When the axes don’t reflect the true range or intervals of the data, it can distort the actual trends, leading to misinterpretation.
For example, if you’re presenting temperature changes over the seasons but your axis isn’t calibrated properly, it can make it seem like the temperature fluctuates wildly when it’s actually more stable.
Here are some tips for ensuring accurate axes scales:
- Check data range: Ensure your axes encompass the full range of your data. If your data points lie between 10 and 50, your scales shouldn't start at 0. Adjust the minimum and maximum values accordingly.
- Use appropriate intervals: Rather than jumping in increments that feel convenient, choose intervals that make sense for the data. For temperature, intervals of 5 or 10 degrees might make the most sense, while financial data might require smaller increments.
- Label axes clearly: Good labeling can eliminate confusion. Display units next to the numbers. If you are measuring distance in kilometers, write it down on the axis.
By diligent checks on both data overlap and axis accuracy, users can ensure their data visualizations convey the right narrative. Always remember, the goal is to create a clear and precise representation of data that improves understanding and insight.
Comparing Line Graphs with Other Graph Types
When diving into the realm of data visualization, it becomes essential to understand how different graph types serve various purposes. Comparing the line graph with other graph types not only clarifies when to choose one over the other but also aids in ensuring the most effective presentation of data. Each type clarifies certain aspects of data differently—providing unique insights to the viewer.
When assessing the suitability of various charts, you’ll find that not all visualizations communicate information in the same way. While line graphs are excellent for displaying trends over time, they sometimes fall short in other contexts. This section outlines the nuances between line graphs, bar graphs, and scatter plots, highlighting their applicability, advantages, and considerations.
Bar Graphs
Bar graphs serve as a useful alternative when you need to compare discrete categories rather than continuous data. They effectively show differences in magnitude among various groups, making them ideal for showing competition or comparisons in observations.
- Visual Representation: Bar graphs utilize vertical or horizontal bars to represent values. Each bar corresponds to a category, making it straightforward to see which category has the highest or lowest value.
- Key Usage: If the primary objective is to compare quantities side by side, bar graphs shine. For example, if you're analyzing survey results about product preferences among different age groups, bar graphs provide a clear visual comparison.
- Limitations: However, bar graphs do not effectively communicate changes over time. When examining how a number has fluctuated over a period, line graphs would be the go-to choice instead.
It's worth considering using bar graphs when the data is categorical, as shown:
"A picture is worth a thousand words. With bar graphs, no need for jargon; the visuals speak volumes."
Scatter Plots
Scatter plots, on the other hand, are a powerful method for displaying relationships between two quantitative variables. They can illustrate how much one variable is affected by another, offering insights that can be pivotal in analytical contexts.
- Data Distribution: Each point in a scatter plot corresponds to a two-dimensional coordinate, revealing patterns, correlations, or clusters. For instance, a scatter plot could depict the relationship between hours studied and scores achieved in an exam, allowing for quick analysis of trends.
- Identifying Correlation: A significant advantage of scatter plots is their ability to demonstrate correlation and causation. Unlike line graphs—which imply a continuous relationship—scatter plots visually present data points, providing insight into data variability.
- Downsides: However, if the goal is to emphasize a progression or trend over time, scatter plots may not be the best option. They are often better suited for exploratory data analysis rather than presentations where trends need clear emphasis.
Epilogue
In this article, we've navigated the intricate process behind creating line graphs, recognizing their pivotal role in data representation. Understanding the nuances not only enhances clarity in your visual data narratives but also aids in informed decision-making. The journey from data collection to graph creation is not a mere sequence of steps; it’s a thoughtful exercise that, when executed well, greatly amplifies the communication of complex ideas.
Emphasizing key considerations, such as maintaining data integrity and choosing appropriate scales, ensures your graphs are not just visually appealing but also mathematically accurate. Moreover, acknowledging the differences between line graphs and other visual tools, like bar graphs and scatter plots, presents a well-rounded perspective that can enrich your analytical toolkit.
Beyond mere aesthetics, line graphs can convey trends and insights that might otherwise slip through the cracks of verbal or textual explanations. They offer a snapshot of data over time or across categories, inviting the audience to draw conclusions swiftly.
Ultimately, honing these skills reinforces your ability to make data-driven decisions. The advantages don’t merely stop at creating a graph; they extend into a greater understanding of the trends and patterns reflecting in your data.