Essential Books to Initiate Your Data Science Journey: A Beginner's Guide
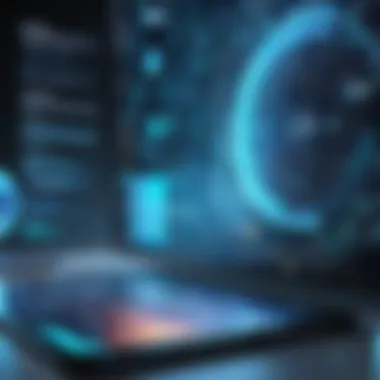
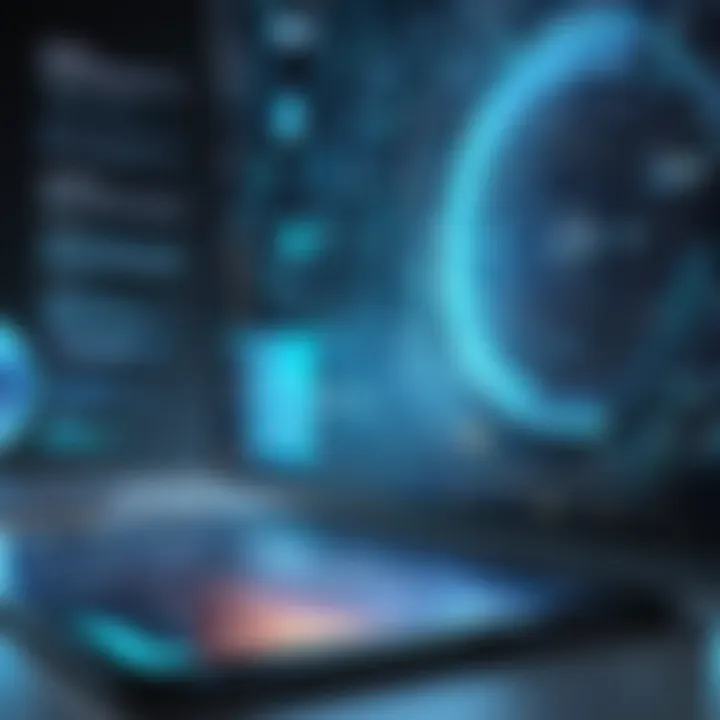
Book Overview
For those embarking on a journey into the intricate realm of data science, the role of essential books cannot be overstated. These literary treasures serve as guiding lights for beginners, illuminating the path towards a profound understanding of data science concepts and principles. By delving into carefully curated books, individuals new to the field can lay a solid foundation and equip themselves with the knowledge necessary to navigate the complexities of data science with confidence and acumen.
Key Books for Beginners
The selection of essential books for beginners in data science is crucial, as it sets the tone for the entire learning process. These books not only introduce fundamental concepts but also present them in a clear and approachable manner, tailored to the needs of novice learners. From the exploration of data analytics to the intricacies of machine learning and artificial intelligence, each book encapsulates valuable insights and practical knowledge, offering a comprehensive overview of the multifaceted field of data science.
Essential Resources
Amidst the vast sea of data science literature, certain books stand out as indispensable resources for beginners. These carefully curated resources cover a wide array of topics, including statistical analysis, programming languages, and data visualization, providing learners with a well-rounded education in the core areas of data science. By immersing oneself in these essential texts, beginners can cultivate a solid understanding of key concepts and methodologies, paving the way for further exploration and specialization within the field.
Practical Applications
Beyond theoretical knowledge, the recommended books for beginners in data science also emphasize practical application and real-world relevance. By incorporating case studies, hands-on exercises, and practical examples, these books bridge the gap between theory and practice, enabling learners to not only grasp complex concepts but also apply them in practical scenarios. This experiential learning approach not only enhances understanding but also fosters critical thinking and problem-solving skills essential for success in the dynamic field of data science.
Conclusion
Introduction to Data Science
Data science plays a crucial role in today's digital landscape, where data-driven decision-making is paramount. Understanding the basics of data science is essential for anyone looking to harness the power of data. In this article, we will delve deep into the core concepts that underpin the field. From defining data science to exploring its applications across various industries, we will unravel the complexity of this dynamic field.
Understanding the Basics
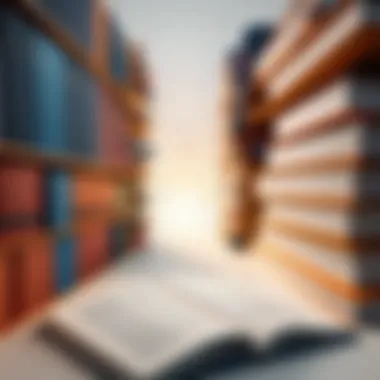
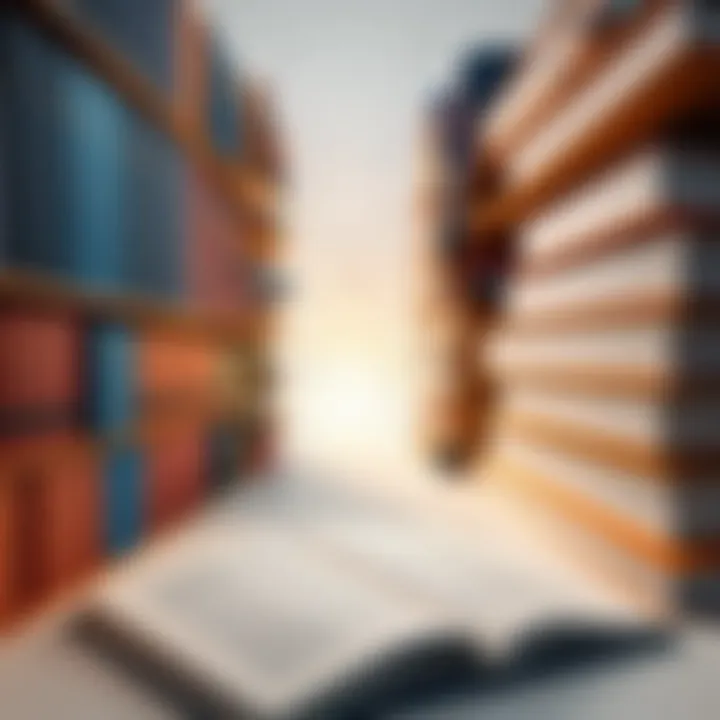
Definition of Data Science
The definition of data science lies at the heart of our exploration. It encompasses the interdisciplinary field that uses scientific methods, algorithms, and systems to extract knowledge and insights from structured and unstructured data. The key characteristic of data science is its ability to translate raw data into tangible outcomes, aiding businesses in making informed decisions. By understanding the intricacies of data science, beginners can gain a solid foundation for advanced learning in the field.
Significance in Today's Context
The significance of data science in today's context cannot be overstated. With the proliferation of data across various sectors, the need for data scientists has surged. Data science empowers organizations to optimize processes, enhance decision-making, and drive innovation. Its interdisciplinary nature makes it a highly sought-after skill set in the digital era. Embracing data science allows individuals to stay relevant in a fast-evolving technological landscape.
Applications in Various Industries
Data science finds applications in diverse industries, including finance, healthcare, marketing, and more. Its ability to uncover trends, patterns, and correlations within data sets revolutionizes how businesses operate. By leveraging data science techniques, organizations can streamline operations, improve customer experiences, and predict future outcomes. Understanding the applications of data science across industries can provide beginners with insights into the real-world impact of their learning journey.
Role of Books in Learning
Impact of Reading on Skill Development
The impact of reading books on skill development is a fundamental aspect of learning data science. Books serve as reservoirs of knowledge, offering in-depth exploration of theoretical concepts and practical applications. By immersing oneself in data science literature, beginners can enhance their analytical thinking, problem-solving skills, and domain expertise. Reading books acts as a catalyst for skill development, paving the way for a comprehensive understanding of the subject.
Benefits of Learning through Books
Learning through books offers numerous benefits for individuals venturing into the realm of data science. Books provide a structured learning path, guiding beginners from foundational concepts to advanced topics. They offer a comprehensive overview of key principles, algorithms, and best practices in data science. By engaging with books, aspiring data scientists can deepen their knowledge, cultivate a holistic perspective, and stay updated on industry trends.
Complementing Academic Knowledge
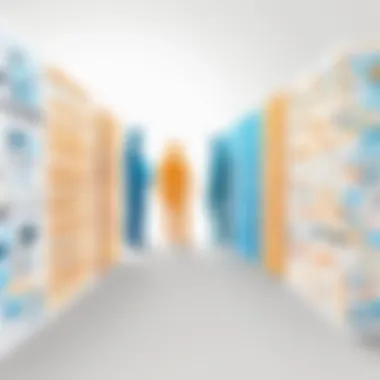
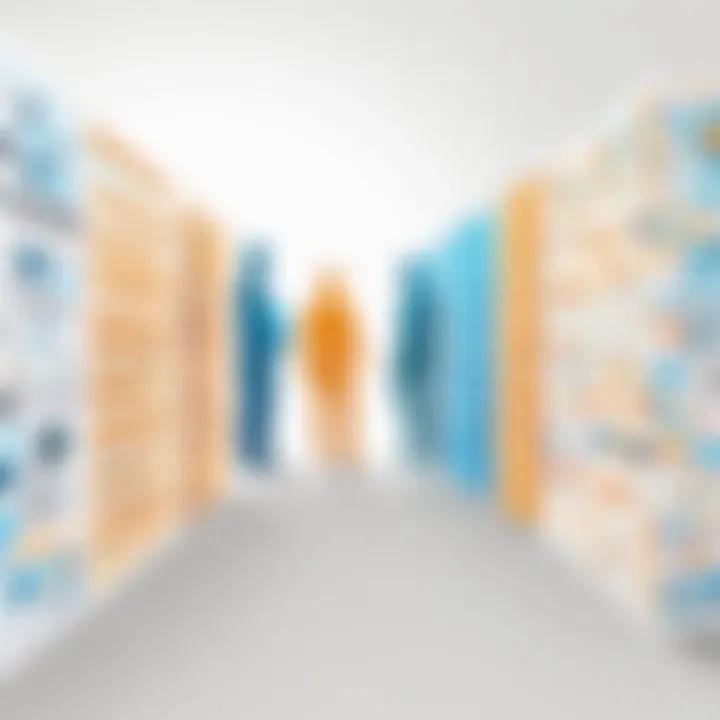
Books play a pivotal role in complementing academic knowledge in data science education. While formal education provides a structured framework for learning, books offer additional insights, practical examples, and case studies that enrich the learning experience. They bridge the gap between theoretical learning and hands-on application, allowing individuals to reinforce their understanding of complex concepts. By incorporating books into their learning curriculum, students can enhance their academic knowledge and practical skills in data science.
Top Books for Beginners in Data Science
In the realm of data science, the selection of books holds paramount importance for beginners embarking on this journey. Choosing the right books can provide a solid foundation and guide individuals through the complex terrain of data science. These books serve as beacons of knowledge, offering insights and techniques essential for honing skills in this field. When considering the top books for beginners in data science, factors such as clarity of concepts, practical applications, and comprehensive coverage of fundamental topics become significant.
Foundational Texts
"Data Science for Beginners" by John Smith
"Data Science for Beginners" by John Smith encapsulates a comprehensive introduction to data science, catering specifically to novices in the field. This book effectively simplifies complex concepts, making it accessible to readers with varying levels of expertise. Its structured approach to presenting foundational principles equips beginners with a strong understanding of key concepts such as data analysis, statistics, and machine learning. The practical examples and exercises integrated within the text enhance the learning experience, allowing readers to apply theoretical knowledge to real-world scenarios.
"Introduction to Data Analytics" by Jane Doe
"Introduction to Data Analytics" by Jane Doe distinguishes itself as a valuable resource for individuals venturing into the realm of data analytics. The book adeptly navigates through the core principles of data analytics, emphasizing the significance of data interpretation and predictive modeling. With a focus on hands-on learning, this book equips beginners with the necessary tools to delve into data analysis and derive actionable insights. Its systematic approach and practical case studies aid in reinforcing key concepts, making it an indispensable guide for beginners.
"The Data Scientist's Toolbox" by Alan Johnson
"The Data Scientist's Toolbox" by Alan Johnson serves as a practical guide for beginners seeking to develop essential skills in data science. This book delves into the tools and techniques crucial for data manipulation, visualization, and interpretation. By bridging the gap between theoretical knowledge and practical application, Alan Johnson equips beginners with the necessary toolbox to navigate through data science projects effectively. The book's emphasis on implementing various tools within the data science workflow enhances the learning process and fosters a deeper understanding of critical concepts.
Navigating Data Science Resources
In the realm of data science education, knowing how to effectively navigate resources is essential. Beginners embarking on this journey must understand the significance of accessing the right tools and materials to develop their skills and knowledge. Navigating Data Science Resources sets the course for enthusiasts to explore various avenues that can shape their learning experience. From online platforms to networking opportunities, this section aims to provide a comprehensive guide on leveraging resources for optimum growth and understanding in data science.
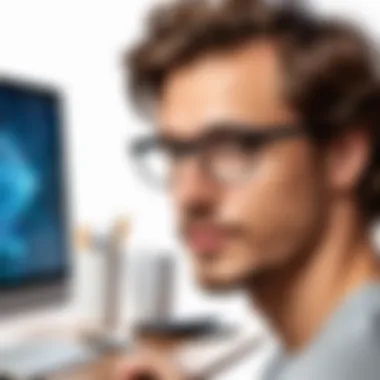
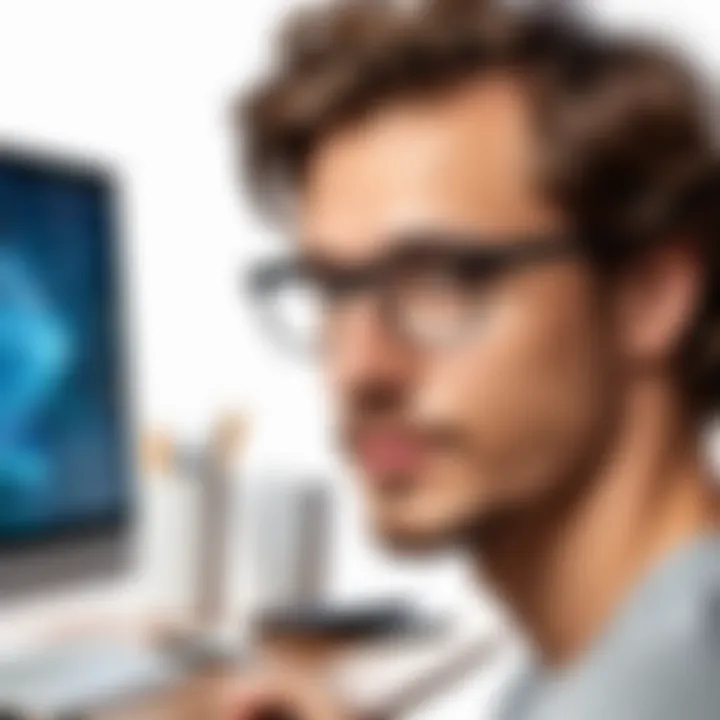
Online Platforms
Utilizing MOOCs for Skill Enhancement
Delving into the world of online learning, Massive Open Online Courses (MOOCs) have emerged as a valuable tool for individuals seeking to enhance their expertise in data science. The appeal of MOOCs lies in their accessibility and flexibility, allowing learners to engage with high-quality course content at their own pace. By harnessing these resources, beginners can acquire essential skills, from programming languages to complex algorithms, laying a solid foundation for their data science journey. While the convenience of MOOCs is undeniable, learners should remain vigilant about the potential pitfalls of self-paced online learning, such as the lack of direct interaction with instructors.
Engaging in Data Science Communities
Another vital aspect of exploring data science resources is active participation in online communities dedicated to the field. Engaging with like-minded individuals in these forums not only fosters a sense of belonging but also provides a platform for knowledge sharing and collaboration. By tapping into data science communities, beginners can seek advice, share insights, and stay abreast of industry trends, enhancing their overall learning experience. However, it is crucial to remain discerning amidst the vast sea of information available in these communities and verify the credibility of sources to ensure reliable and accurate guidance.
Exploring Virtual Labs and Projects
Virtual labs and projects serve as virtual playgrounds for data science enthusiasts to apply theoretical knowledge to practical scenarios. By engaging with these interactive tools, beginners can hone their analytical skills, experiment with different methodologies, and troubleshoot real-world data challenges in a controlled environment. The hands-on experience gained through virtual labs not only reinforces conceptual understanding but also cultivates problem-solving abilities essential for success in data science. While the convenience of virtual labs is undeniable, learners should approach these platforms with a critical mindset to derive maximum benefit from the experience.
Conclusion
In wrapping up the exploration of data science for beginners and essential books to kickstart their journey, it is crucial to emphasize the pivotal role of continuous learning in this field. Continuous learning is not merely an option but a fundamental necessity for those aiming to excel in data science. This aspect stands as the backbone of progress, allowing individuals to stay abreast of the rapidly evolving landscape of data analytics and machine learning. The thirst for continuous learning sets apart successful data scientists from the rest by fostering adaptability, resilience, and a growth mindset. Embracing this ethos ensures that aspiring data professionals keep honing their skills and expanding their knowledge base to achieve mastery in the dynamic realm of data science.
Embarking on Your Data Science Journey
Importance of Continuous Learning
Unveiling the significance of continuous learning within the data science domain unveils its transformative power in cultivating expertise beyond initial training. The cycle of learning never truly ends in data science due to the ever-changing technologies, methodologies, and tools that demand perpetual upskilling. Emphasizing the importance of continuous learning equips beginners with the vital discipline needed to navigate through intricate data sets and complex algorithms. By embracing a learning mindset that thrives on curiosity and experimentation, individuals can push boundaries, unlock hidden insights, and foster innovation within the data science domain. Continuous learning primes beginners for constant growth, enabling them to remain competitive and valuable in a field driven by innovation and discovery.
Exploring Diverse Topics in Data Science
Understanding the relevance of exploring diverse topics in data science sheds light on the holistic approach needed to comprehend the intricacies of this multifaceted field. By delving into diverse topics, beginners gain a comprehensive understanding of the various branches within data science, including machine learning, artificial intelligence, data visualization, and predictive analytics. This exploration not only broadens one's knowledge base but also fosters interdisciplinary connections and insights that can fuel creative problem-solving in real-world scenarios. Immersing oneself in diverse topics encourages a well-rounded skill set, enabling individuals to tackle complex challenges with ingenuity and precision, thus positioning them as versatile data science professionals capable of driving meaningful impact.
Utilizing Resources Effectively
The art of utilizing resources effectively emerges as a critical component in the journey of a budding data scientist, offering a strategic advantage in optimizing learning outcomes. Effectively utilizing resources entails discerning the most relevant and current sources of information, tools, and frameworks that align with one's learning objectives and career aspirations. By leveraging resources thoughtfully, beginners can streamline their learning curve, enhance their problem-solving capabilities, and accelerate their professional growth in the data science domain. Moreover, effective resource utilization fosters efficiency, productivity, and innovation, enabling individuals to make informed decisions, experiment with novel techniques, and elevate their expertise in data science practices for tangible results.