Discover the Top Data Science Books for all Expertise Levels
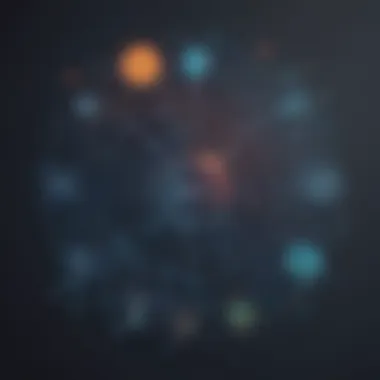
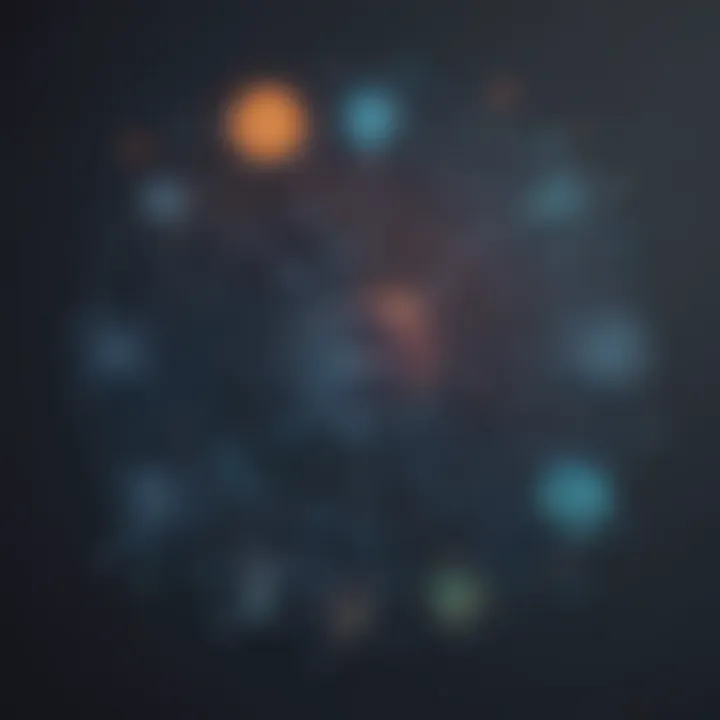
Delve into the realm of data science with our meticulously curated selection of recommended books that span all levels of proficiency. Whether you're a novice looking to grasp the fundamentals or a seasoned expert in search of cutting-edge insights, our guide is tailored to enhance your journey through the lucrative domain of data science.
App Overview
Embark on a transformative expedition across the ever-evolving landscape of data science with our handpicked collection of literature π. Whether you seek a foundational understanding or aspire for specialized knowledge, our guide aims to facilitate your exploration, irrespective of your current expertise level.
In terms of functionalities, our guide offers comprehensive coverage of various aspects, ranging from basic concepts to intricate methodologies. Readers stand to benefit from a diverse array of resources that cater to their individual aspirations within the data science sphere.
Step-by-Step Walkthrough
To begin your data science odyssey, acquaint yourself with the intricacies of our recommended literature. Each book recommendation is accompanied by detailed insights on its content, target audience, and the core concepts it explores. Readers can expect a user-friendly interface that provides easy navigation through the dynamic realm of data science literature.
Visual aids, such as screenshots and illustrations, are incorporated to augment your understanding and assist in visualizing complex ideas presented in various texts. By following our step-by-step instructions, you can efficiently navigate through diverse topics and harness the knowledge embedded within each book for your personal and professional growth.
Tips and Tricks
Accelerate your mastery of data science literature with expert tips tailored to enhance your reading experience and comprehension. Uncover hidden gems within the recommended books, offering invaluable insights that can sharpen your analytical skills and broaden your perspective on data-related concepts.
Explore shortcuts and unique features embedded within the texts that can streamline your learning process and amplify the benefits derived from each reading session. By embracing these tips and tricks, you can extract maximum value from the recommended books and enrich your data science journey.
Common Issues and Troubleshooting
While navigating the expansive realm of data science literature, users may encounter challenges or roadblocks that hinder their learning experience. Our guide is equipped with a dedicated section that anticipates common issues and offers effective troubleshooting techniques to overcome them.
From understanding complex theories to deciphering intricate algorithms, readers can find solutions to perplexing scenarios they may encounter during their exploration of data science literature. By equipping yourself with these troubleshooting strategies, you can ensure a seamless and enriching reading experience that propels your understanding of key data science concepts.
App Comparison
In a market saturated with data science literature options, it is vital to differentiate between various offerings to make an informed decision. Our guide conducts a thorough comparison of recommended books, highlighting their unique selling points, distinguishing features, and areas of expertise.
By contrasting different texts and analyzing their content, readers can pinpoint the most suitable resources that align with their learning objectives and proficiency level. This critical evaluation enables users to make informed decisions when selecting data science literature that best serves their educational and professional needs.
Understanding Data Science Fundamentals
In the realm of data science, grasping the core fundamentals forms the bedrock for any aspiring enthusiast or seasoned professional embarking on this enriching journey. Understanding Data Science Fundamentals provides a strong foundation upon which intricate data concepts and techniques can be built. It serves as the gateway to unlocking the complexities of data analysis, interpretation, and manipulation, essential in harnessing the power of big data effectively. By delving into this topic, readers can expect to gain insight into the underlying principles that govern the field of data science, equipping them with the requisite knowledge to navigate through more advanced concepts and methodologies with confidence.
Introduction to Data Science
Exploring the essence of data science
Delving into the essence of data science unveils a world where data transforms into actionable insights, driving decision-making processes across various industries. This exploration encompasses the art of extracting meaningful information from data sets, uncovering patterns, trends, and correlations that lay the groundwork for informed conclusions and strategic planning. The essence of data science lies in its ability to convert raw data into valuable knowledge, empowering organizations to optimize operations, enhance customer experiences, and innovate solutions. Understanding this fundamental aspect is crucial for individuals aiming to harness the potential of data-driven decision-making and leverage the vast opportunities that data science offers.
Key concepts and terminologies
Key concepts and terminologies form the lexicon of data science, representing the building blocks upon which data analysis and interpretation are structured. From familiarizing oneself with terms like 'predictive modeling' and 'machine learning' to understanding the significance of 'data mining' and 'feature engineering,' mastering these concepts is paramount for effective communication and collaboration within the data science domain. Embracing key terminologies empowers professionals to converse fluently in the language of data, enabling them to dissect complex data processes, methodologies, and outcomes with precision and clarity. It is through a profound understanding of these basic concepts that individuals can navigate through advanced data science literature and practical applications with ease.
Importance of Data Literacy
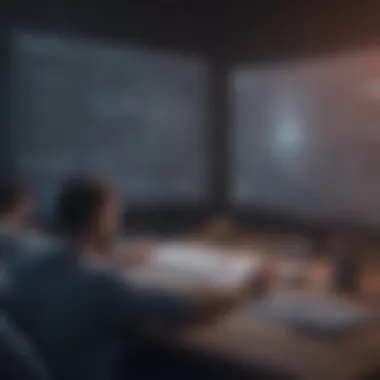
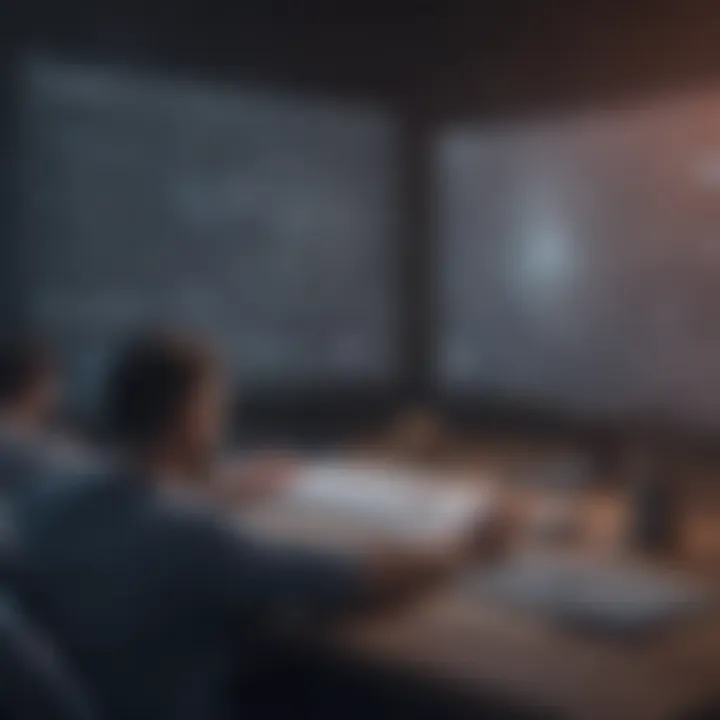
Data literacy stands as a pillar in the realm of data science, underscoring the significance of possessing the skills to interpret, analyze, and derive insights from data effectively. Enhancing data interpretation skills involves honing the ability to extract valuable information from datasets, discern patterns, and derive actionable conclusions that drive strategic decision-making. By developing proficiency in data interpretation, individuals can empower organizations to leverage data as a strategic asset, guiding them in optimizing processes, identifying market trends, and mitigating risks efficiently.
Enhancing data interpretation skills
Improving data interpretation skills equips individuals with the capacity to discern meaningful insights from vast amounts of data, unraveling underlying trends and correlations that potentially impact business operations and performance. By enhancing this skill set, professionals are better positioned to extract actionable intelligence from complex datasets, illuminating pathways for innovation, process optimization, and competitive advantage. Data interpretation skills serve as a catalyst for informed decision-making, enabling organizations to formulate strategies based on empirical evidence rather than conjecture or intuition.
Real-world applications
Real-world applications of data literacy extend beyond the realm of theoretical knowledge, translating data interpretation skills into practical solutions and tangible outcomes. By applying data literacy in real-world scenarios, individuals can drive organizational transformation, optimize operational processes, and uncover new business opportunities through data-driven insights. The value of real-world applications lies in their ability to bridge the gap between theoretical concepts and actionable results, demonstrating the tangible impact of data literacy in driving innovation, informed decision-making, and sustainable growth within organizations.
Mathematics for Data Science
Mathematics serves as the backbone of data science, providing the theoretical framework through which data is analyzed, interpreted, and modeled. Statistical methods play a pivotal role in data analysis, enabling professionals to draw inferences, make predictions, and validate hypotheses based on empirical data. Understanding statistical methods equips individuals with the tools to uncover patterns, trends, and outliers within datasets, facilitating evidence-based decision-making and actionable insights.
Statistical methods
Statistical methods encompass a diverse range of techniques aimed at analyzing, interpreting, and presenting data in a meaningful format. From descriptive statistics that summarize and describe data distributions to inferential statistics that draw conclusions and make predictions based on sample data, these methods form the crux of data analysis and interpretation. By mastering statistical methods, individuals can unveil hidden patterns within datasets, establish causal relationships, and derive empirical insights that drive strategic decision-making processes within organizations.
Linear algebra essentials
Linear algebra serves as the mathematical foundation for advanced data science techniques such as machine learning, deep learning, and predictive modeling. Understanding linear algebra essentials enables professionals to manipulate data matrices, perform dimensionality reduction, and optimize computational algorithms for data analysis. From solving systems of linear equations to eigenvector decomposition and singular value decomposition, these essentials underpin the sophisticated mathematical operations inherent in data science applications, empowering individuals to tackle complex data problems with mathematical rigor and precision.
Essential Data Science Books for Beginners
In the realm of data science exploration, understanding the basics is paramount, making essential data science books for beginners a cornerstone of knowledge acquisition. These foundational resources provide an entry point for novices, offering simplified concepts and practical examples that serve as building blocks for more advanced learning. Beginners stand to benefit significantly from embracing these fundamental texts, as they lay a robust groundwork for future expertise development. Considering essential data science books for beginners ensures a structured learning path, equipping individuals with the necessary tools to comprehend complex data science principles.
Data Science for Dummies
Simplified concepts for beginners
Delving into the realm of simplified concepts for beginners within data science for dummies unravels a treasure trove of foundational knowledge. These simplified concepts break down intricate topics into digestible bits, aiding beginners in grasping complex theories with ease. The key characteristic of simplified concepts lies in their ability to bridge the gap between abstract data science theories and practical application, offering a clear learning trajectory. This makes them a popular choice for individuals seeking a structured approach to data science exploration. The unique feature of simplified concepts lies in their simplification without compromising essential knowledge, striking a delicate balance that accelerates beginners' learning journeys.
Practical examples
Exploring practical examples within the scope of data science for dummies enriches beginners' understanding through hands-on applications. Practical examples serve as real-world demonstrations of theoretical concepts, enabling learners to apply their knowledge in relevant scenarios. The key characteristic of practical examples is their ability to contextualize theoretical information, translating abstract theories into tangible outcomes. This aspect makes practical examples a beneficial choice for this article, as they enhance comprehension and retention of data science principles through practical application. The unique feature of practical examples is their demonstration of the direct relevance of data science theories in solving practical problems, providing hands-on experience to learners.
Python for Data Science Handbook
Fundamentals of Python programming
Within the Python for Data Science Handbook, the exploration of fundamentals of Python programming offers a robust foundation for data science enthusiasts. Understanding the principles of Python programming is essential for data manipulation and analysis, making it a crucial choice for this article. The key characteristic of Python programming fundamentals is their versatility and simplicity, allowing beginners to grasp essential programming concepts efficiently. This aspect makes it a popular choice for individuals embarking on their data science journey. The unique feature of Python programming lies in its extensive library support and readability, enhancing coding efficiency and promoting streamlined data analysis within the data science context.
Data manipulation techniques
Delving into data manipulation techniques within the Python for Data Science Handbook uncovers a realm of essential skills for data processing and analysis. These techniques enable practitioners to transform raw data into valuable insights, facilitating informed decision-making. The key characteristic of data manipulation techniques is their capability to enhance data processing efficiency, enabling users to derive meaningful conclusions from complex datasets. This attribute makes data manipulation techniques a beneficial choice for this article, as they empower individuals with the skills to extract valuable insights from data. The unique feature of data manipulation techniques lies in their versatility and applicability across diverse data science domains, providing a versatile toolkit for analyzing data effectively.
The Data Science Handbook
Insights from industry experts
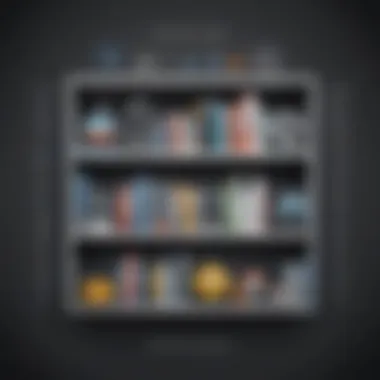
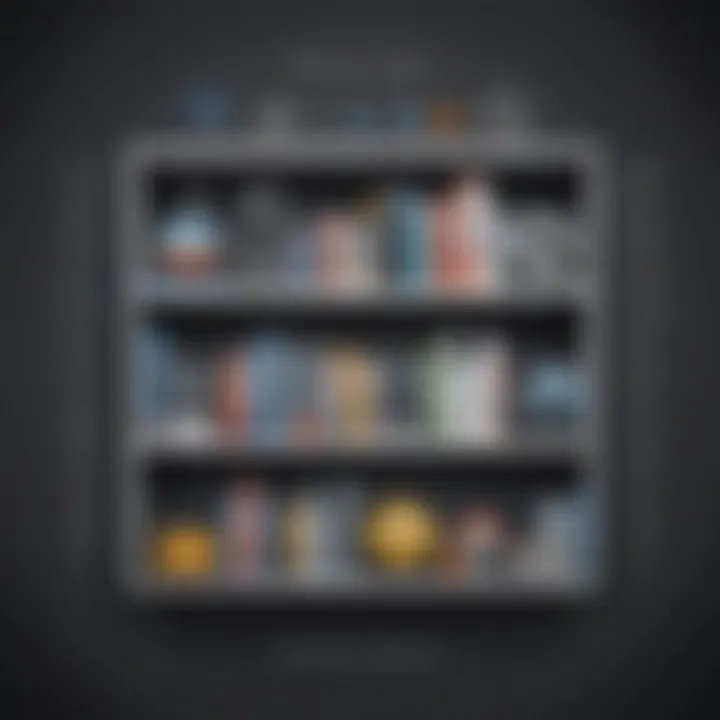
Exploring insights from industry experts within The Data Science Handbook unveils a wealth of knowledge curated from experienced professionals. These insights offer valuable perspectives on real-world data science applications, enriching readers' understanding of industry best practices. The key characteristic of insights from industry experts is their practical relevance and alignment with industry standards, making them a valuable choice for this article. The unique feature of insights from industry experts lies in their provision of insider tips and strategies for navigating the dynamic landscape of data science, offering expert guidance to aspiring data science practitioners.
Career guidance
Navigating career guidance within The Data Science Handbook provides individuals with essential advice and strategies for advancing their professional journeys. This career guidance covers various aspects, such as skill development, networking, and career paths within the data science field. The key characteristic of career guidance is its tailored approach to individual career aspirations, offering personalized insights to enhance career progression. This aspect makes career guidance a beneficial choice for this article, as it equips readers with practical tools and strategies for succeeding in the data science industry. The unique feature of career guidance is its strategic focus on long-term career growth, empowering professionals to make informed decisions and pursue rewarding opportunities.
Advanced Data Science Literature
In this section of the article, we delve into the realm of Advanced Data Science Literature, an indispensable component of our curated guide to the best data science book recommendations. Advanced Data Science Literature plays a pivotal role in equipping readers with in-depth knowledge and insights essential to mastering the complexities of data science. By exploring advanced topics and cutting-edge techniques, readers can elevate their understanding and skills in this dynamic field, paving the way for career growth and innovation.
Machine Learning Yearning by Andrew Ng
Advanced Machine Learning Concepts
Diving into the specifics of advanced machine learning concepts presented in Andrew Ng's seminal work, we encounter a treasure trove of sophisticated algorithms and methodologies designed to tackle intricate data science challenges. The emphasis on intricate models and algorithms empowers readers to grasp complex patterns within data, fostering a deeper appreciation for the nuances of machine learning. The unparalleled depth of coverage on advanced topics ensures that readers are equipped with the arsenal needed to excel in a competitive data science landscape, making this book a prized gem in our recommended list.
Optimizing Model Performance
Within the realm of optimizing model performance lies a critical aspect of data science that cannot be overlooked. By fine-tuning models and enhancing predictive accuracy, practitioners can unlock the true potential of their data analysis efforts. The book's dedicated focus on optimizing model performance not only underscores the importance of refining predictive models but also offers strategies and techniques to streamline this process effectively. Understanding how to enhance model performance is imperative in data science, making this facet a valuable addition to our comprehensive guide.
Deep Learning by Ian Goodfellow
Deep Dive into Neural Networks
Delve into the intricacies of neural networks with Ian Goodfellow's exploration of deep learning. Through a detailed examination of neural network architectures and processes, readers gain a profound understanding of how these structures operate and contribute to the field of data science. The book's nuanced approach to portraying neural networks as the foundation of deep learning elucidates their significance in modern-day data analysis, making it an indispensable resource for enthusiasts seeking to demystify this complex domain.
Cutting-Edge Research Applications
By shedding light on cutting-edge research applications, Ian Goodfellow broadens the horizons of data science enthusiasts, offering a glimpse into the future possibilities of this evolving field. Through real-world examples and case studies, readers can witness the transformative potential of innovative research applications in driving data science advancements. This unique perspective on the intricate interplay between research and practical applications distinguishes Ian Goodfellow's work as a pioneering source of knowledge in the realm of deep learning, positioning it as a must-read for aspiring data scientists.
Practical Statistics for Data Scientists
Statistical Analysis in Data Science
The foundation of robust data science lies in sound statistical analysis, a core tenet expertly explored within Practical Statistics for Data Scientists. By dissecting statistical methods and concepts essential for data analysis, this book equips readers with the analytical tools required to derive meaningful insights from data sets. The comprehensive overview of statistical analysis not only clarifies intricate statistical techniques but also underscores their pivotal role in driving informed decision-making in data science. Leveraging statistical analysis skills is imperative for data scientists, making this facet of the book a vital component in our recommended reading list.
Practical Case Studies
Embark on a journey through practical case studies that exemplify the real-world applications of statistical analysis in data science. By immersing readers in concrete scenarios and data sets, Practical Statistics for Data Scientists bridges the gap between theoretical knowledge and practical application, fostering a holistic understanding of statistical analysis. The emphasis on hands-on learning and problem-solving instills a practical mindset in readers, empowering them to tackle complex data challenges with confidence. Through detailed case studies, readers can glean valuable insights and methodologies, enriching their data science repertoire and advancing their analytical prowess.
Specialized Data Science Topics
Data Visualization Mastery
Visualizing data insights effectively
Visualizing data insights effectively is a crucial aspect of data science that allows individuals to present complex information in a clear and understandable manner. By using visual representations such as charts, graphs, and dashboards, data scientists can communicate their findings more efficiently, enabling data-driven decision-making processes. The key characteristic of visualizing data effectively lies in its ability to simplify complex datasets, making patterns and trends easily discernible. This approach is highly beneficial for our article as it emphasizes the importance of data visualization in comprehending and conveying significant insights. Additionally, the unique feature of visualizing data effectively is its capacity to engage and resonate with readers, enhancing their understanding of the data presented while minimizing cognitive load.
Tools and techniques
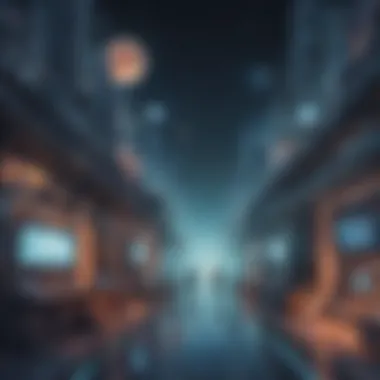
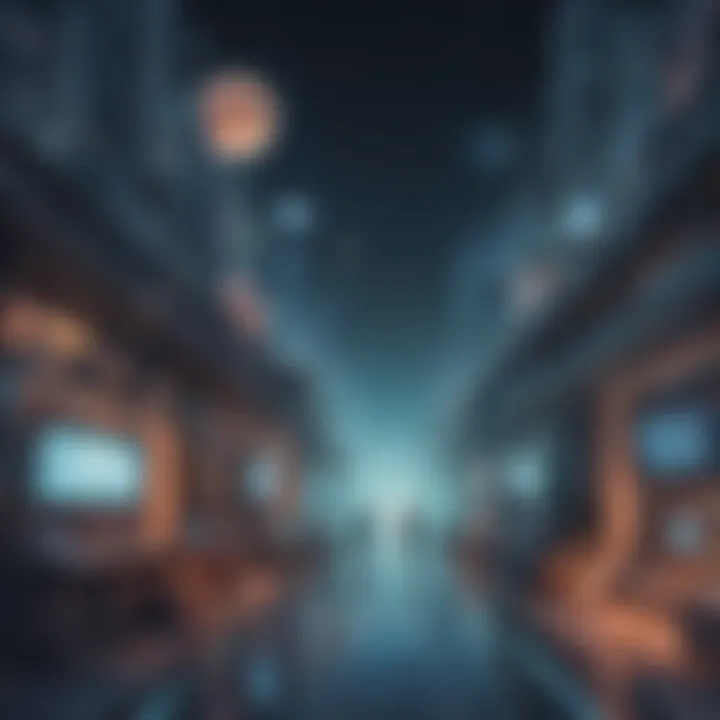
Discussing tools and techniques in data visualization is pivotal as it provides readers with valuable resources to create impactful visual representations of data. Highlighting key software such as Tableau, Power BI, and Python libraries like Matplotlib and Seaborn can empower readers to explore diverse visualization methods efficiently. The key characteristic of leveraging these tools and techniques is the seamless integration of data sources to produce compelling visual narratives. These resources are popular choices for our article due to their user-friendly interfaces and robust capabilities in generating interactive and insightful visualizations. While these tools offer immense advantages in enhancing data comprehension, potential disadvantages may include a learning curve associated with mastering these platforms, a factor that readers should consider when adopting them for data visualization projects.
Big Data Analytics Handbook
Handling vast datasets efficiently
Efficiently handling vast datasets is a critical component of data science, especially in the era of big data. This aspect focuses on techniques and strategies to manage, process, and extract valuable insights from massive amounts of data. The key characteristic of this capability lies in its scalability and performance, allowing data scientists to analyze and derive meaningful conclusions from voluminous datasets effectively. This choice is beneficial for our article as it sheds light on the importance of optimizing data processes to handle increasing data volumes efficiently. Furthermore, the unique feature of handling vast datasets efficiently is its capacity to streamline data operations, resulting in time and cost savings for organizations.
Scalable analytics solutions
Discussing scalable analytics solutions contributes significantly to our article by highlighting advanced methods to manage and analyze extensive datasets seamlessly. Scalable analytics solutions offer the flexibility and agility required to adapt to evolving data needs, ensuring that organizations can derive actionable insights efficiently. The key characteristic of these solutions is their ability to process massive amounts of data rapidly without compromising accuracy or performance. Their popularity in our article stems from their effectiveness in addressing complex data challenges and facilitating informed decision-making. While these solutions offer numerous advantages in enhancing data analytics capabilities, potential disadvantages may include the complexity of implementation and the need for specialized expertise to leverage these tools effectively.
Ethical Considerations in Data Science
Ensuring data privacy and security
Emphasizing the importance of ensuring data privacy and security in data science is crucial to maintaining trust and integrity in data-driven practices. This aspect focuses on implementing measures and protocols to safeguard sensitive information, prevent unauthorized access, and adhere to data protection regulations. The key characteristic of ensuring data privacy and security lies in establishing secure data handling procedures and ethical data practices, ensuring the confidentiality and integrity of information. This choice is beneficial for our article as it underscores the ethical obligations and responsibilities associated with handling data ethically and responsibly. Additionally, the unique feature of ensuring data privacy and security is its capacity to mitigate risks associated with data breaches and unauthorized data access, safeguarding organizations and individuals from potential harm.
Ethical decision-making frameworks
Discussing ethical decision-making frameworks adds a critical perspective to our article by exploring ethical guidelines and principles that govern data science practices. These frameworks provide a structured approach for data scientists to navigate complex ethical dilemmas and make informed decisions that align with moral and legal standards. The key characteristic of ethical decision-making frameworks is their emphasis on transparency, accountability, and fairness in data-related activities, promoting ethical conduct and integrity in data science endeavors. This choice is valuable for our article as it promotes ethical awareness and encourages readers to uphold ethical standards in their data practices. Furthermore, the unique feature of ethical decision-making frameworks is their adaptability to diverse contexts and scenarios, enabling data scientists to make ethically sound decisions in challenging situations.
Next Steps in Your Data Science Journey
In this section of our article, we delve deep into the pivotal topic of 'Next Steps in Your Data Science Journey,' emphasizing its crucial role in guiding individuals through the vast landscape of data science. Understanding the importance of continuous learning and progression is essential for anyone looking to excel in this field. By offering insights into the specific elements, benefits, and considerations surrounding the advancement in data science, we aim to equip our readers with the necessary tools to navigate their journey effectively.
Continuous Learning in Data Science
Exploring emerging trends
Exploring emerging trends plays a significant role in shaping the data science landscape, driving innovation, and staying ahead of the curve. By discussing the latest advancements, technologies, and methodologies, individuals can gain a comprehensive understanding of the industry's trajectory. The key characteristic of exploring emerging trends lies in its ability to provide fresh perspectives and insights, allowing practitioners to adapt and evolve in a fast-paced environment. This emphasis on staying informed about emerging trends is a valuable choice for our article as it equips readers with up-to-date knowledge and fosters a proactive approach towards continuous learning. The unique feature of exploring emerging trends is its capacity to enhance decision-making processes and inspire novel ideas, although it may require dedication and consistent effort to remain informed.
Engaging with the data science community
Engaging with the data science community is instrumental in fostering collaboration, sharing knowledge, and expanding one's professional network within the field. By participating in community forums, attending industry events, and engaging with like-minded individuals, practitioners can cultivate a supportive environment for growth and learning. The key characteristic of engaging with the data science community lies in its encouragement of knowledge exchange and peer-to-peer learning, creating opportunities for mentorship and collaboration. This aspect is a beneficial choice for our article as it promotes a sense of community among readers and facilitates ongoing discussions and insights. The unique feature of engaging with the data science community is its potential to expose individuals to diverse perspectives and experiences, although it may require active participation and communication skills to fully leverage its advantages.
Practical Application of Data Science Knowledge
Hands-on projects and collaborations
Hands-on projects and collaborations offer a practical avenue for applying theoretical knowledge, honing skills, and gaining real-world experience in data science. By engaging in hands-on projects, individuals can test their understanding, experiment with different techniques, and enhance their problem-solving abilities. The key characteristic of hands-on projects and collaborations is their emphasis on experiential learning and skill development, providing a platform for individuals to grow and showcase their capabilities. This aspect is a popular choice for our article as it encourages active participation and hands-on learning, fostering a deeper understanding of theoretical concepts. The unique feature of hands-on projects and collaborations is their ability to simulate real-world scenarios and challenges, although they may require time and dedication to execute effectively.
Building a robust portfolio
Building a robust portfolio is essential for establishing credibility, showcasing expertise, and attracting potential opportunities in the data science field. A well-structured portfolio that highlights one's projects, achievements, and contributions can set individuals apart and demonstrate their skills to prospective employers or collaborators. The key characteristic of building a robust portfolio is its role in personal branding and self-promotion, creating a lasting impression on viewers and validating one's proficiency in data science. This choice is beneficial for our article as it emphasizes the importance of showcasing practical work and achievements, adding depth and credibility to one's professional profile. The unique feature of building a robust portfolio is its potential to open doors to new career prospects and collaborations, although it may require ongoing maintenance and updates to remain relevant.
Career Advancement Strategies
Navigating career paths in data science
Navigating career paths in data science involves strategic planning, continuous learning, and adaptability to exploit diverse opportunities within the field. By mapping out potential trajectories, setting clear goals, and acquiring relevant skills, individuals can progress towards their desired roles and outcomes. The key characteristic of navigating career paths in data science is its emphasis on self-awareness and exploration, empowering individuals to make informed decisions and navigate challenges effectively. This aspect is a popular choice for our article as it offers readers practical insights and tools to structure their career trajectories in data science. The unique feature of navigating career paths in data science is its potential to spark self-reflection and goal-setting, although it may require perseverance and flexibility to navigate the dynamic job market.
Professional development tips
Professional development tips are invaluable resources for enhancing skills, expanding knowledge, and fostering personal growth within the data science domain. By following expert advice, staying updated on industry trends, and investing in continuous learning, individuals can elevate their professional capabilities and remain competitive in the job market. The key characteristic of professional development tips is their focus on actionable strategies and best practices, offering readers practical guidance to enhance their career prospects. This aspect is a beneficial choice for our article as it provides concrete advice and recommendations for career advancement in data science. The unique feature of professional development tips is their ability to inspire self-improvement and success, although they may require commitment and implementation to yield tangible results.
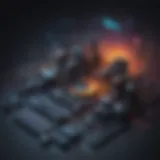
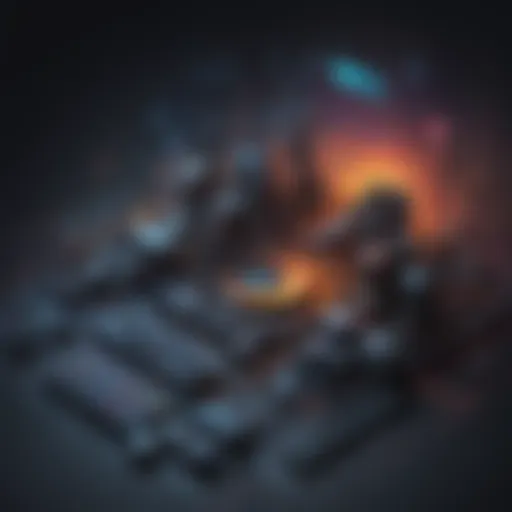