Exploring Behavioral Analytics and Its Impact
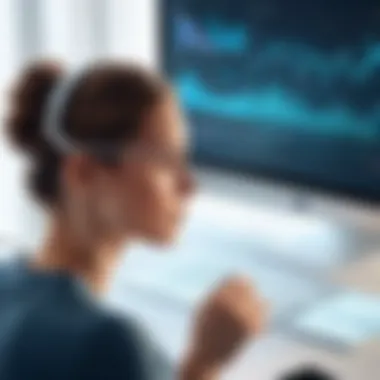
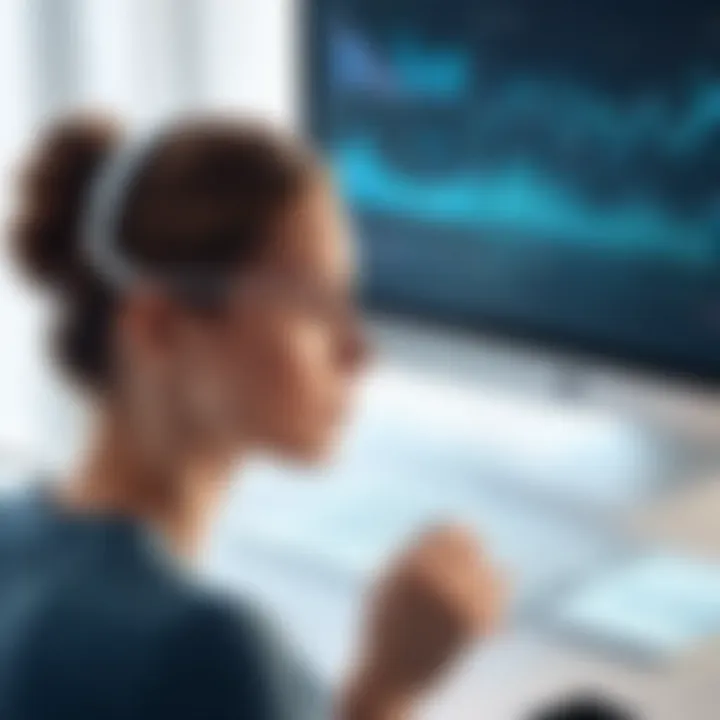
Intro
Behavioral analytics has gained momentum in recent years, transforming the landscape of how we interpret and respond to user actions. This burgeoning field involves the extraction and analysis of data regarding user behavior, aiding businesses and developers in crafting more intuitive experiences. Understanding behavioral analytics is pivotal; it not only sheds light on the intricacies of audience engagement but also empowers organizations to tailor their strategies effectively.
At its core, behavioral analytics revolves around observing patterns and trends in user behavior. By leveraging this data, businesses can pinpoint what makes users tick, how they interact with different interfaces, and ultimately how to enhance satisfaction and retention. The implications stretch far and wide, influencing marketing approaches, application development, and even customer service tactics. In fact, being well-versed in behavioral analytics can set a clear roadmap for future innovations.
The application of these insights allows organizations to secure their competitive edge, adapting swiftly to the changing needs and preferences of their users.
"Understanding your users' behavior is the key to creating products that resonate with them."
With that said, let’s embark on a journey through the multifaceted domain of behavioral analytics. We'll dive into its significance, dissect methodologies, and uncover how its principles are applied across various fields ranging from e-commerce to app development. This exploration aims to highlight not just the insights one can gain, but also the ethical considerations and emerging trends that shape the discussion around responsible data use.
Through this lens, we can appreciate how the delicate balance between harnessing data and maintaining user privacy continues to shape the narrative within the tech community. Let's turn the page and explore the foundational elements of behavioral analytics.
Foreword to Behavioral Analytics
In today's fast-paced digital landscape, understanding user behavior has become paramount for organizations aiming to stay ahead. Behavioral analytics serves as a bridge between raw data and decision-making, offering insights into how users interact with products and services. It's not just about collecting data but interpreting it effectively. This section aims to lay the groundwork for a comprehensive exploration into what behavioral analytics encompasses and why it has become an essential element in various industries.
Defining Behavioral Analytics
Behavioral analytics is the process of aggregating data generated by users' interactions with technology. This includes everything from the clicks on a website to the duration spent on a particular page. The goal is to analyze this data to identify patterns that can inform future decisions. By employing various analytical techniques, organizations can make sense of complex data sets and draw conclusions about user preferences, needs, and behaviors.
This approach is distinct from traditional analytics, which often only focuses on aggregated metrics like total page views or transactions. Behavioral analytics digs deeper, aiming to uncover the "why" behind the data. For instance, why do users abandon shopping carts? What features keep users coming back? Understanding these nuances allows businesses to tailor their products and services, leading to enhanced customer satisfaction and loyalty.
Historical Context of Behavioral Data
The notion of gathering data about user behavior isn't as modern as it seems. While technology has revolutionized how we collect and analyze data, the roots can be traced back to market research methods of the early 20th century. Initially, businesses relied on surveys and focus groups to gauge customer preferences.
With the advent of the internet, especially in the late 1990s and early 2000s, consumer behavior data began to flourish. Online activity started to be logged through cookies and tracking codes, providing a wealth of material for analysis. The e-commerce boom further propelled this trend, as businesses recognized the substantial advantage of understanding online consumer behavior.
As technology continued to evolve, so did the methods of data collection and analysis. Today, organizations can leverage advanced machine learning algorithms to derive insights from behavioral patterns that are so intricate they were once unfathomable. As a result, behavioral analytics not only aids businesses in optimizing their strategies but also enriches user interactions in ways that were previously unimaginable.
The Importance of Behavioral Analytics
Behavioral analytics is more than just a buzzword tossed around in marketing meetings. It’s a powerful tool that’s reshaping how businesses interact with their audiences. By diving into user behaviors, companies glean insights that can significantly impact their operational strategies and ultimately drive growth. In an era defined by technological advances, the importance of understanding behavioral patterns cannot be overstated. Here’s a closer look at why behavioral analytics matters.
Impact on Decision Making
In the world of business, making decisions based on gut feelings or assumptions often leads businesses down a path of trial and error. However, behavioral analytics provides organizations with concrete data on how users engage with products or services. This leads to more informed decision-making.
For instance, an e-commerce site analyzing user clicks can pinpoint which products are the most popular, informing inventory decisions. Similarly, tracking how often a customer abandons their cart can uncover potential friction points in the purchasing process.
- Data-Driven Decisions: Organizations that leverage behavioral analytics experience a significant shift in how they approach challenges. Using data rather than intuition reduces the risk of costly mistakes.
- Trend Identification: By observing patterns and trends in user behavior, companies can adapt quickly to changes in consumer preferences. This agility not only retains current customers but also attracts new ones.
- Risk Mitigation: Behavioral insights can flag potential issues before they escalate. If analytics reveal a steep decline in user engagement, teams can dive deeper to figure out the root causes and tackle them head-on.
"Analyzing user behavior is like having a compass; it directs your strategies where they matter most."
Enhancing User Experience
User experience (UX) has become a central pillar in app development and web design. A seamless UX not only improves user satisfaction but also drives conversion rates. Behavioral analytics plays a critical role in tailoring experiences that meet user expectations.
When users interact with a product, they leave behind digital footprints that can be analyzed. For example, a mobile app might track how users navigate through various features. If analytics show many users struggle at a certain step, it would prompt the development team to reassess and improve that aspect of the app.
- Personalization: Behavioral analytics enables a degree of personalization that was previously unattainable. Users appreciate tailored experiences, like recommendations based on previous purchases, enhancing engagement.
- User Testing and Feedback: Utilizing tools to gather feedback helps refine products. Analyzing this data alongside behavioral metrics helps to ensure modifications are beneficial and resonate with users.
- Improved Retention: By understanding what keeps users engaged or drives them away, businesses can implement strategies that promote long-term user loyalty.
In summary, the importance of behavioral analytics cannot be diminished. From making astute decisions to enhancing user experience, it stands as a transformative force that can redefine how businesses operate and connect with their consumers.
Key Components of Behavioral Analytics
Behavioral analytics is not just a buzzword; it's a comprehensive approach to understanding user interactions and decision-making processes through data. The key components of this field act as the backbone for any analytic strategy. Understanding these elements is crucial for anyone keen on decoding user behavior, whether in marketing, product development, or even user experience design.
Data Collection Methods
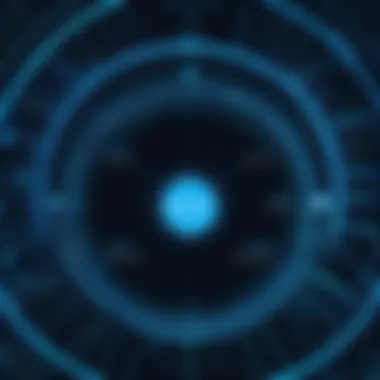
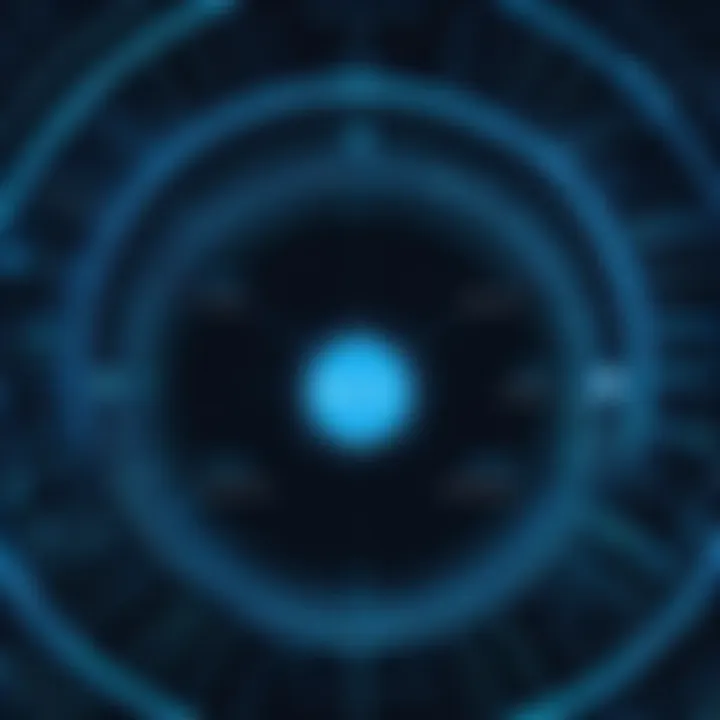
User Interaction Tracking
User interaction tracking is all about capturing the dynamics of how users engage with digital platforms. It's like having a behind-the-scenes pass to every click, scroll, and hover a user makes. This granularity allows businesses to identify patterns in behavior that might otherwise go unseen.
One of the hallmark characteristics of user interaction tracking is its ability to provide real-time insights. Companies can immediately see which features are popular and which ones might be causing frustration. This immediacy not only assists in quickly addressing issues but also informs future design decisions.
However, there are some downsides. The sheer volume of data collected can be overwhelming, leading to analysis paralysis if not handled correctly. Additionally, privacy concerns loom large, as users might feel their every move is scrutinized.
Surveys and Feedback Tools
Surveys and feedback tools offer a more direct line to user sentiment, capturing thoughts and feelings in a way that numbers alone cannot. By soliciting opinions, businesses can gather qualitative insights that complement quantitative data obtained through tracking.
One major advantage here is that surveys can target specific aspects of user experience. For example, a well-timed survey asking about a particular feature's usability can provide immediate feedback when it is most relevant. This targeted approach can unveil insights that even the most thorough tracking might miss.
On the flip side, surveys can suffer from low response rates and bias, as users who feel strongly (positively or negatively) are more likely to respond. Crafting well-designed surveys can counter this, but it requires effort and expertise.
Analytics Techniques
Descriptive Analytics
Descriptive analytics serves as the starting line for understanding data. It summarizes past behaviors and trends to give you a clear view of what has happened. This technique is instrumental in identifying patterns over time, like seasonal spikes in user engagement or the most visited pages on an app.
By utilizing descriptive analytics, businesses can tailor their strategies based on what has been effective in the past. However, it can't predict future behavior, which limits its scope in guiding proactive decisions. The insights from this form of analysis are invaluable, providing a solid foundation for further analytical pursuits.
Predictive Analytics
Predictive analytics is where things start to get interesting. Using historical data, it aims to forecast future behavior or trends. Imagine being able to predict which users are likely to churn before they actually do. This method employs machine learning algorithms and statistical techniques to recognize patterns that may not be apparent with descriptive analytics.
While predictive analytics can be a game changer, it does rely heavily on the quality and completeness of the historical data collected. Without reliable data, predictions can go awry, leading to misguided strategies.
Prescriptive Analytics
Prescriptive analytics takes predictive insights a step further. It's not just about what could happen; it's about recommending actions based on those predictions. This technique provides actionable insights, helping organizations devise strategies that align with anticipated outcome.
For instance, if predictive analytics identifies potential churn in a segment of users, prescriptive analytics can suggest specific interventions to retain them. This dual approach can optimize decision-making but may require advanced tools and expertise to implement effectively.
As behavioral analytics evolves, the key components outlined above will play a pivotal role in shaping user interactions, ultimately enhancing the overall experience in various domains.
Applications of Behavioral Analytics
Behavioral analytics has become a cornerstone for various sectors. It unlocks a treasure trove of data-driven insights, allowing businesses and developers to tailor their strategies effectively. By examining how users interact with products and services, companies can mold their offerings to better meet customer needs. This relevance becomes particularly pronounced in fields like marketing, product development, and user retention. The applications of behavioral analytics not only refine strategies but also open avenues for innovation.
In Marketing Strategies
Customer Segmentation
Customer segmentation is a key aspect of marketing that greatly benefits from behavioral analytics. By using data to understand different user categories based on their behaviors, companies can effectively target specific groups. This method enables businesses to craft tailored messages that resonate with distinct customer segments.
A standout feature of customer segmentation is its ability to personalize marketing efforts. Instead of taking a one-size-fits-all approach, organizations can concentrate their resources on segments that demonstrate higher engagement potential. The unique characteristic of this approach lies in its ability to deliver content that aligns with users’ preferences and habits. This makes it a popular choice for marketers aiming to boost conversion rates.
However, there are challenges that come with this. One might misinterpret data or overlook smaller segments that could have a larger potential. Being aware of these disadvantages is key to using customer segmentation effectively.
Personalized Marketing Campaigns
Personalized marketing campaigns are an extension of customer segmentation and take it a step further. They aim to deliver unique customer experiences through tailored messages and incentives. The effectiveness here stems from using users’ past behaviors to predict future actions.
The core advantage of personalized marketing lies in its capacity to enhance user engagement and loyalty. Campaigns designed specifically for individual preferences are likely to yield a deeper connection between the brand and the user. A unique feature of this approach is its reliance on real-time data to adjust marketing strategies quickly, which is beneficial in a fast-paced digital environment. Yet, a potential downside is that constant personalization efforts may come off as intrusive or overwhelming for some users.
In Product Development
User-Centric Design
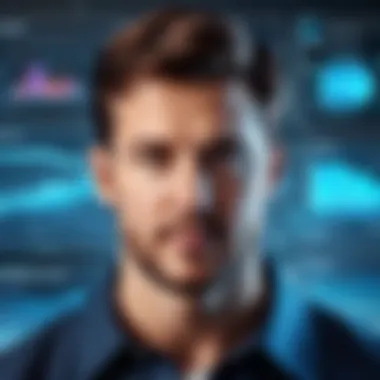
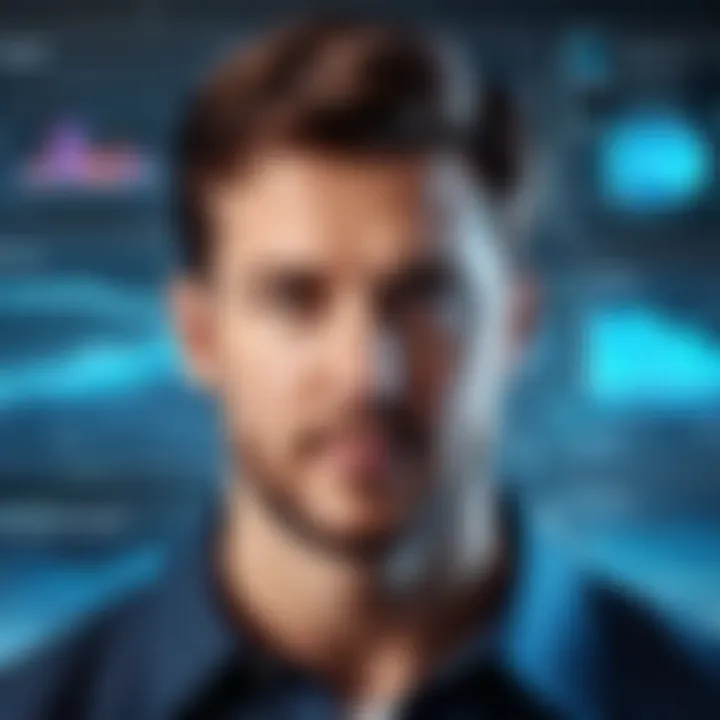
User-centric design is where behavioral analytics shines in product development. This involves developing products that prioritize user needs and feedback. Understanding users' interactions can inform design choices significantly, ensuring that the end product aligns with user expectations.
An essential trait of user-centric design is the iterative process it utilizes. By continuously integrating user feedback, developers can refine and enhance their products over time. This dynamic adaptability is a major reason for its popularity. One unique aspect is that it places users at the forefront of the design process, ensuring satisfaction at every release stage. However, being excessively reactive to user feedback might lead to design fatigue, where constant changes could confuse users instead of engaging them.
Feature Usability Testing
Feature usability testing is crucial for ensuring that products are intuitive and meet user needs effectively. This process allows developers to gather specific insights on how users interact with new features before full deployment. Through behavioral analytics, teams can identify friction points and usability issues that users might face.
A notable advantage of this approach is that it significantly lowers the risk of product failure upon launch. By testing features early, teams can implement necessary changes and enhancements. Its unique aspect allows for real-time user feedback, which ensures that the final product is user-tested and approved. On the flip side, overemphasis on usability testing might delay product launches, hindering speed to market.
In User Retention
Churn Prediction Models
Churn prediction models harness behavioral analytics to identify users likely to disengage. By interpreting user behavior patterns, these models can provide insights into when and why users may leave, allowing companies to take proactive measures.
The characteristic power of churn prediction lies in its proactive nature. Instead of waiting for users to disengage, companies can act swiftly, offering incentives or addressing issues before the user decides to leave. A unique feature is the integration of machine learning, which enhances the accuracy of predictions over time. However, the complexity of developing these models can also pose challenges, requiring a balance of technical knowledge and user understanding.
Retention Strategies
Retention strategies are critical for maintaining a loyal customer base. These strategies often stem from insights gleaned through behavioral analytics, allowing companies to craft tailored programs aimed at improving user retention.
A key characteristic of effective retention strategies is their adaptability; they evolve based on ongoing analysis of user behavior. By focusing on what keeps users engaged, businesses can foster lasting relationships with their audience. It's a beneficial choice, particularly in competitive markets where customer loyalty is paramount. That said, there’s a risk that misapplied retention tactics can feel artificial or forced, potentially alienating users rather than fostering loyalty.
"The application of behavioral analytics is a critical component of modern marketing and product strategy, offering insights that drive innovation and enhance user interaction."
Challenges in Behavioral Analytics
Behavioral analytics offers powerful insights into user behavior, yet it is not without its challenges. Addressing these hurdles is essential for organizations to fully leverage the benefits of this analytical approach. In this section, we will delve into various challenges, including data privacy issues, accuracy concerns, and the intricacies of interpreting complex data patterns. Understanding these challenges equips businesses and app developers with the knowledge to navigate the complexities inherent in this field.
Data Privacy Issues
Data privacy has emerged as a hot-button issue in today's digital landscape. With the increasing amount of data being collected, businesses often find themselves walking a tightrope. On one end, there's the value derived from detailed user data; on the other, the potential backlash from breaches or misuse.
In recent years, high-profile data breaches have heightened awareness around data security. Users are more cautious about who they share their information with. Organizations must prioritize transparency regarding data use, seeking explicit consent and ensuring compliance with regulations, such as the General Data Protection Regulation (GDPR) in Europe.
Moreover, it’s crucial to implement robust security measures. This includes encrypting sensitive data, regularly updating security protocols, and training employees on data protection principles. Failure to address these concerns could lead to loss of consumer trust and expensive legal repercussions.
Data Accuracy Concerns
Accuracy in data collection is paramount in behavioral analytics. Inaccurate or incomplete data can result in misguided insights, ultimately leading to poor decision-making. This challenge arises from various sources, including user errors during data entry, technical glitches, or biases in data algorithms.
For instance, consider a scenario involving an app that tracks user preferences. If there’s an error in how data is recorded (for example, a misinterpretation of user clicks), the insights drawn will not accurately reflect user behavior. Consequently, businesses might invest time and resources into marketing strategies based on faulty data.
To mitigate these concerns, organizations should perform regular audits of their data collection methods. Implementing validation checks and employing data cleansing techniques can enhance accuracy. By ensuring high-quality data, businesses can derive insights that drive effective strategies and improve user experiences.
Interpreting Complex Data Patterns
Data collected is often intricate and multifaceted. Users’ behaviors are influenced by a confluence of factors, making it tricky to draw straightforward conclusions. For instance, a user may interact with an app in multiple ways, influenced by factors such as time of day, personal mood, or external events like sales or promotions.
This complexity means that interpreting data patterns requires a nuanced approach. Relying solely on trend analysis might lead to oversimplification. Instead, it’s essential to employ sophisticated tools and techniques, such as machine learning, to unearth hidden patterns and relationships within the data. Machine learning models can learn from historical data, identify trends, and even predict future behavior based on complex variables.
Additionally, collaboration among team members—data scientists, product managers, and UX designers—can help elucidate complex findings. Engaging in interdisciplinary discussions fosters a richer understanding of user behavior and can assist in formulating strategies that resonate on various levels.
"The greatest challenge is not the data itself, but how we choose to interpret and utilize it."
Ethical Considerations in Behavioral Analytics
In the fast-paced world of behavioral analytics, one cannot merely skim the surface without addressing the deeper ethical implications that govern its use. It’s not just about what can be measured, but rather how and why those measurements are taken. The importance of ethical considerations in this field transcends mere compliance; it extends into the realm of trust and responsibility towards users. As app developers and tech-savvy professionals leverage behavioral data, they walk a fine line between maximizing insights and preserving user dignity.
Understanding these ethical dimensions can lead to not only better practices but also stronger relationships with users. When organizations prioritize ethics, they not only comply with regulations but also cultivate a loyal user base.
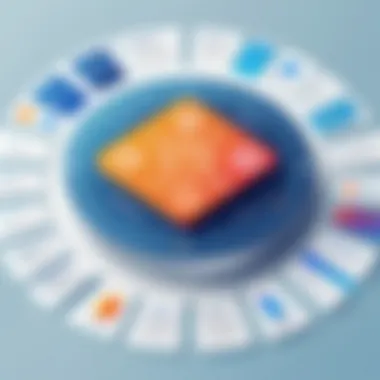
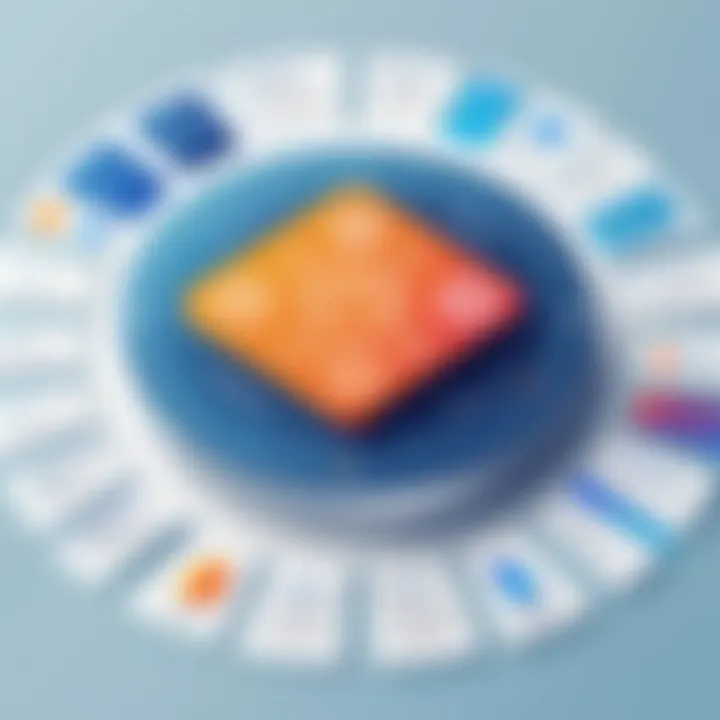
Informed Consent and Transparency
Informed consent stands as a cornerstone of ethical behavioral analytics. Simply put, users ought to know what data is being collected, how it is being used, and the potential implications of that data usage. After all, it's their behavior—or more importantly, their data—that is at the heart of the analysis.
Imagine visiting a new app for the first time. You scroll through the terms and conditions and are met with a wall of text—none of which makes sense in a real-world context. This lack of transparency breeds confusion and distrust. Developers must strive to communicate clearly, breaking down complex jargon into digestible nuggets that users can easily understand. Misleading users, even unintentionally, can lead to reputational damage and potential legal repercussions.
The benefits of transparency are manifold. Firstly, it empowers users, giving them agency over their data. Secondly, it establishes a relationship built on trust, which can lead to higher engagement levels. Finally, it reduces the likelihood of regulatory scrutiny, preventing costly fines and setbacks. It's essential to create user-friendly privacy notices and implement features that allow users to adjust their data preferences effortlessly, establishing a good practice of informed consent.
Bias in Data Interpretation
Bias in data interpretation poses a significant threat in behavioral analytics. Data, while ostensibly objective, is often prone to the biases of those who collect and analyze it. This can skew insights and lead to misguided conclusions. For instance, if a development team predominantly consists of individuals from one demographic, their interpretations may inadvertently reflect that group's perspectives, sidelining the experiences of others.
To mitigate these risks, organizations should establish diverse teams that bring various perspectives to the table. Employing people with different backgrounds and experiences can enhance the critical analysis of data patterns. Using methods such as algorithmic audits can also help identify potential biases within models.
Moreover, creating a culture of continuous learning is vital. Reminding everyone involved that they must regularly hone their skills in interpreting data responsibly can go a long way. Regular training sessions focused on ethical data practices can ensure that teams remain vigilant against biases.
"The greatest danger in times of turbulence is not the turbulence; it is to act with yesterday's logic." — Peter Drucker
As behavioral analytics continues to evolve, so too must the ethical frameworks that guide it. Emphasizing informed consent and addressing bias are not just ethical imperatives; they are necessary steps for building a sustainable future in this domain. Without these considerations, the proliferation of behavioral analytics runs the risk of becoming a double-edged sword, potentially undermining user trust and damaging the reputation of businesses.
Future Trends in Behavioral Analytics
In the world of behavioral analytics, staying one step ahead is not just advantageous; it is essential. As technology progresses at a breakneck pace, the interplay between user behaviors and data analytics continually shapes industries. Businesses and developers who fine-tune their understanding of these future trends can harness powerful insights that promote strategic decision-making and foster user engagement. As we traverse this section, expect to uncover the increasing relevance of behavioral data, and examine specific advancements that will play pivotal roles in the coming years.
Integration with Artificial Intelligence
Artificial Intelligence (AI) is setting the stage for a vast overhaul in how behavioral analytics evolves and operates. The integration of AI into analytics systems presents numerous benefits that can no longer be overlooked. Machine learning models have matured, capable of sifting through colossal data sets—something that would take humans an eternity to accomplish. The ability to analyze user interactions, preferences, and even hesitations, with surgical precision, helps carve a path toward personalized user experiences.
Moreover, AI-driven tools can adapt to changing user behaviors in real-time. For instance, e-commerce platforms using advanced AI algorithms can predict customer preferences based on past purchases and even suggest products before users realize they need them. This not only enhances the user experience but also increases conversion rates dramatically.
Considerations:
- Scalability: AI allows companies to analyze data at scale without the need for proportional increases in manpower.
- Responsiveness: Improved algorithms will enable businesses to respond promptly to trends as they unfold.
- Bias Mitigation: While AI is not immune to biases present in the data it's trained on, efforts aimed at refining algorithms are underway to promote fairness and inclusiveness in data interpretation.
"AI and behavioral analytics together can create a symphony of insights, making information not just informative, but transformative."
Evolution of Predictive Analytics
Predictive analytics isn't merely an analytical technique; it's the crystal ball of data. Over recent years, we have seen this discipline evolve significantly. The trajectory points toward more sophisticated methodologies that leverage historical data to predict future behaviors. Companies that adapt to these enhancements will find themselves better equipped to anticipate customer needs and refine their strategies accordingly.
Today's predictive models utilize advanced techniques that incorporate neural networks and natural language processing. With these tools in their arsenal, businesses can derive insights from unstructured data sources like emails, social media, and customer reviews. For instance, analyzing user sentiments from social media posts can unearth trends that guide product improvement or customer service enhancements.
The future will likely see predictive analytics becoming even more integrated within operational frameworks, leading to a shift from merely understanding what has happened to proactive decision-making.
Key benefits include:
- Enhanced Accuracy: Predictive models will become more precise as they evolve, minimizing the margin of error in forecasts.
- Proactive Strategies: Organizations will be able to preemptively address issues before they escalate.
- Cross-Discipline Applications: Insights gained from predictive analytics will likely influence various areas, from sales forecasting to resource allocation and beyond.
Closure
In wrapping up our extensive examination of behavioral analytics, it is vital to underscore the multifaceted elements this area encompasses. The insights drawn throughout this article elucidate the crucial role behavioral analytics plays in various sectors, especially in informing decision-making, enhancing user experiences, and steering marketing strategies. This importance stems not merely from the data collected but from how such data is interpreted and applied.
Summarizing Insights
Behavioral analytics offers a wealth of understanding about user engagement and preferences. By leveraging methods like user interaction tracking and predictive modelling, organizations can craft tailored experiences that resonate with individuals. This synergy between data and business strategy equips companies to be more responsive to the evolving landscape of consumer behavior. Moreover, with a focus on strategy refinement grounded in solid analytics, businesses can allocate resources more effectively and anticipate customer needs in a timely manner.
- Deep Insights into User Behavior: Behavioral analytics enables businesses to analyze patterns which in turn helps in modifying services or products to better align with user desires.
- Data-Driven Decision Making: With continual evaluation of user actions, companies can make informed choices instead of relying solely on intuition.
- Competitive Edge: Staying ahead means adapting quickly; behavioral analytics provides that edge by highlighting emerging trends and user feedback swiftly.
"The future belongs to those who prepare for it today." - Malcolm X
Call to Action for Future Research
As we conclude, it's clear that the journey into behavioral analytics is only just beginning. The fast-paced evolution of technology demands ongoing research and development in this field. There are several pathways for future exploration:
- Ethical Frameworks: With privacy concerns at the forefront, creating robust frameworks that address data ethics will be essential.
- Integration with Machine Learning: Investigating how advanced algorithms can enhance predictive analytics and data interpretation can open new avenues for insights.
- Cross-Industry Applications: Behavioral analytics can be adapted beyond tech companies; seeing its application in healthcare, education, and other industries might provide enriching perspectives.
In summary, the quest for understanding human behavior through data is a dynamic and promising field. As we look forward, the importance of keeping pace with emerging trends cannot be overstated. Let this signal the beginning of further inquiries, innovative projects, and collaborative efforts to harness the power of behavioral analytics.